Can AI ever completely avoid having hallucinations? This question continues to intrigue researchers and poses a significant challenge to the widespread acceptance of artificial intelligence (AI) models.
Hallucinations, referring to the generation of inaccurate information or nonsensical text, have been a persistent issue plaguing large language models like ChatGPT since their introduction to the public.
Recognizing this problem, researchers have been actively working to address and improve the situation, but finding a foolproof solution remains elusive.
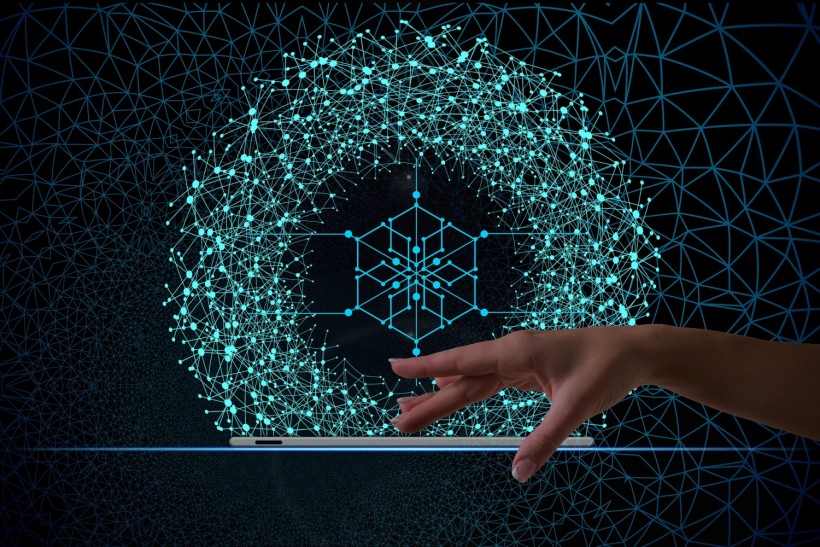
What Are AI Hallucinations?
AI hallucinations refer to situations where artificial intelligence systems, particularly language models, generate outputs that contain false or inaccurate information. These hallucinations occur due to how AI models are trained and their limitations in understanding context and real-world knowledge.
When AI models are trained, they are exposed to vast amounts of data from various sources, including the internet. This data contains a mix of accurate and inaccurate information.
During the training process, the AI system learns patterns and associations in the data to generate human-like text in response to queries or prompts.
However, AI models lack genuine comprehension and reasoning abilities like humans. They rely solely on the patterns they have learned from the training data.
As a result, when faced with queries that fall outside their training data or context, they may produce responses that are plausible-sounding but factually incorrect.
Read Also: Copyright Issues Are Prevalent in AI Models Like ChatGPT, New Study Claims
Experts Weight In
In an interview with Fox News Digital, Kevin Kane, CEO of American Binary, a quantum encryption company, emphasized the current lack of complete understanding of how machine learning arrives at its conclusions.
The "black box nature" of AI algorithms makes it difficult to fully comprehend and eliminate hallucinations. Making significant changes to the way AI models work may be necessary, but that, in turn, raises the question of how feasible such an approach is.
Christopher Alexander, the chief analytics officer of Pioneer Development Group, discussed the idea of a "use case" and its implications for AI models. He explained that AI's ability to handle various topics and situations can lead to varying issues with hallucinations.
While some problems might be fixable, expecting to solve every challenge in AI development might be unrealistic. Alexander advocated for a case-by-case approach, where researchers document the issues and work towards improvements.
Emily Bender, a linguistics professor and director of the Computational Linguistics Laboratory at the University of Washington, highlighted the "inherent" mismatch between technology and its intended use case. This mismatch arises due to researchers attempting to apply AI to multiple scenarios, which can lead to challenges in eliminating hallucinations entirely.
In some cases, AI developers may opt to repurpose existing models to address specific problems rather than building entirely new models from scratch. Although this approach might not yield perfect results, it can be a starting point for further refinements and customizations.
Alexander noted that circumstances and requirements can vary significantly from one case to another, suggesting that refining AI models for specific tasks or industries may be a more effective approach in the long run.
Related Article: AI Is Being Used in Dating Apps to Create Witty Opening Lines and Appealing Profiles