A group of researchers at the University of Waterloo have introduced a novel artificial intelligence (AI) model designed to enhance trustworthiness and accuracy in AI-generated decisions while addressing bias concerns.
According to the team, conventional machine learning models frequently produce biased outcomes, favoring larger population groups or being influenced by hidden variables, and detecting these biases can be a laborious process involving deciphering intricate patterns across different categories or primary sources.
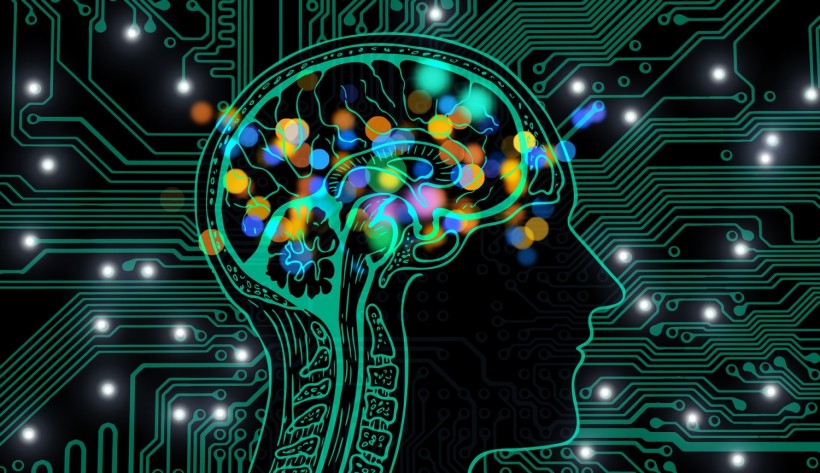
Biased AI in the Medical Sector
The medical sector particularly faces serious implications from biased machine learning outputs. Healthcare practitioners heavily rely on extensive medical datasets and complex algorithms to make critical judgments regarding patient care.
Machine learning streamlines data organization, saving time. However, this approach may miss patient groups with uncommon symptomatic patterns, leading to misdiagnoses and unequal healthcare consequences for certain individuals.
Under the leadership of Dr. Andrew Wong, a professor emeritus of systems design engineering at the University of Waterloo, a new model has been developed to tackle these challenges.
This innovative model is designed to disentangle intricate data patterns and correlate them with specific root causes unaffected by anomalies or mislabelled instances, thereby enhancing trust and reliability in Explainable Artificial Intelligence (XAI).
"This research represents a significant contribution to the field of XAI," noted Wong, adding that the team was able to unveil physicochemical amino acid interaction patterns hidden in protein binding data from X-ray crystallography.
These patterns were previously obscured due to the interweaving of multiple factors in the binding environment. According to Wong, the disentanglement of these entangled statistics provided a more accurate representation of deeper knowledge embedded in the data.
XAI refers to a subset of AI and machine learning techniques designed to provide understandable and interpretable explanations for the decisions and predictions made by AI systems.
In other words, XAI aims to make the inner workings of AI models more transparent and understandable to humans. The team's breakthrough paved the way for the development of the Pattern Discovery and Disentanglement (PDD) model.
Read Also: Biochip Technology Enables Swift Detection of Cancer Protein Markers, Viruses
The PPD Model
Dr. Peiyuan Zhou, the lead researcher on Wong's team, highlighted the model's mission to bridge the gap between AI technology and human comprehension, thus, enabling dependable decision-making and unearthing profound insights from complex data sources.
According to the team, the PDD model has brought about a revolution in the realm of pattern discovery. Several instances from diverse case studies have highlighted PDD's capacity to anticipate medical outcomes for patients using their clinical records as a basis.
Additionally, the PDD system can unearth fresh and uncommon patterns within datasets, enabling researchers and practitioners to identify mislabeled data points or anomalies within machine learning processes.
The findings suggest that healthcare experts can now provide more dependable diagnoses underpinned by strong statistical evidence and easily understandable patterns. This, in turn, aids in enhancing treatment suggestions for various diseases across different stages.
The team's findings were further detailed in the journal npj Digital Medicine.
Related Article: Medical Professionals Are Unprepared for Ai's Involvement in Healthcare, Study Reveals