Employing the power of artificial intelligence, a research team delves into the realm of dam management, revolutionizing the approach to optimize operations and predict behaviors. In response to the growing impact of climate challenges on Korea's dams, this innovative use of deep learning not only forecasts patterns but unveils the decision-making intricacies guiding these vital structures.
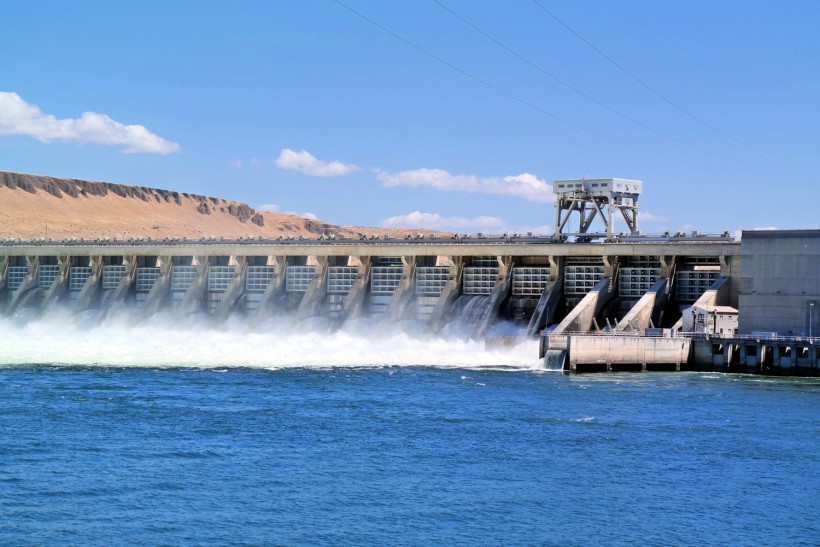
Using AI to Monitor Dams
A research team led by Professor Jonghun Kam and Eunmi Lee, a Ph.D. candidate from the Division of Environmental Science & Engineering at Pohang University of Science and Technology (POSTECH), has recently leveraged deep learning techniques to analyze dam operation patterns and evaluate their effectiveness, as reported in Tech Xplore.
In August 2020, a dam near the Seomjin River in Korea faced overflow and incurred damages exceeding USD 76 million due to a combination of prolonged drought and intense rainfall.
This incident prompted the research team to explore whether predictive dam management could have averted such disasters. Korea heavily relies on dams and associated infrastructure for water management, especially during the summer precipitation peak.
However, the global climate crisis has led to unpredictable weather events such as typhoons and droughts, posing challenges for dam operations. Hence, the research team embarked on a study aiming to enhance conventional physical models by employing an AI model trained on extensive big data.
The team aims to develop an AI model capable of predicting the operational patterns of dams within the Seomjin River basin, specifically the Seomjin River Dam, Juam Dam, and Juam Control Dam. Additionally, they wanted to understand the decision-making processes of the trained AI models.
Gated Recurrent Unit
Utilizing the Gated Recurrent Unit (GRU) model, a deep learning algorithm, the team trained it with data spanning from 2002 to 2021 from dams along the Seomjin River. Input variables included precipitation, inflow, and outflow data, while hourly dam levels served as outputs.
The team claims that the analysis achieved impressive accuracy, with an efficiency index surpassing 0.9.
The team then created scenarios to understand how the trained GRU model responded to alterations in inputs, such as -40%, -20%, +20%, and 40% changes in each input variable. The study revealed that while changes in precipitation had a minimal impact on dam water levels, variations in inflow significantly impacted the water level.
Interestingly, the identical change in outflow resulted in different water levels at distinct dams, indicating that the GRU model had learned the unique operational nuances of each dam. Professor Jonghun Kam explained that the examination went beyond predicting dam operation patterns to assess their effectiveness using AI models.
The team introduced a methodology to indirectly understand the decision-making process of AI-based black box models determining dam water levels.
"Our aspiration is that this insight will contribute to a deeper understanding of dam operations and enhance their efficiency in the future," Professor Jonghun Kam said in a statement.
The findings of the study were published in the Journal of Hydrology.
Related Article: Oneka Technologies Unveils Wave-Powered Desalination Machine: A Solution to Increasing Global Water Scarcity?