For years, researchers in artificial intelligence and robotics have been striving to develop versatile embodied intelligence or agents capable of performing tasks in the real world with the same agility and understanding as animals or humans.
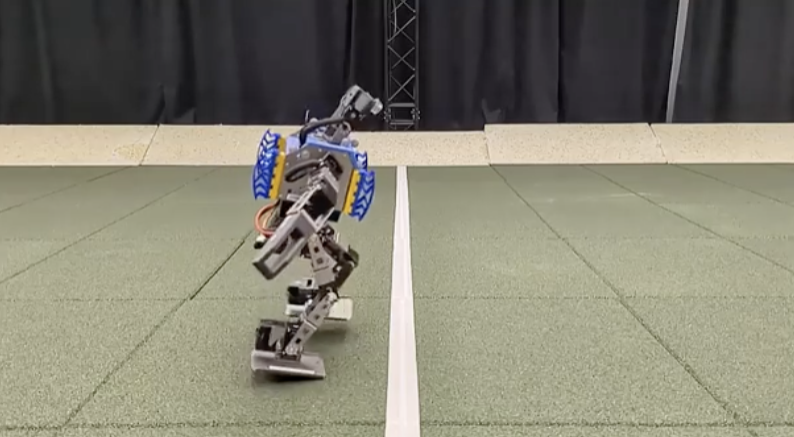
Exploring the Potential of Mini Soccer Robots Trained
A mini soccer robot powered by artificial intelligence falls, then quickly returns to dribble and score. In matches, these robots, trained using deep reinforcement learning, showed unexpected behaviors like pivoting and spinning, which are hard to program in advance.
Researchers used small humanoid robots trained through deep reinforcement learning to demonstrate their agility and skill in one-on-one soccer matches. As published in Science Robotics, the robots showcased their mobility by walking, turning, kicking, and recovering swiftly after falls.
They smoothly switched between different actions and learned to predict ball movement and defend against their opponents' shots as they played strategically. Based on findings from Google's DeepMind team, deep reinforcement learning could offer a method to train humanoid robots in fundamental and reliable movements.
Advancements in deep reinforcement learning have propelled recent progress in this endeavor. While quadrupedal robots have demonstrated various skills, such as movement, handling objects, and manipulation, controlling humanoid robots has been more challenging.
This is primarily due to stability and hardware limitations, resulting in a focus on basic skills and reliance on model-based predictive control. The team adopted this method to train affordable, easily accessible robots for multi-robot soccer, exceeding expected levels of agility.
Training Mini Soccer Robots for Agility, Adaptability
They showcased the robots' ability to control their movements based on sensory input in both simulated environments and real-world situations, focusing on simplified one-on-one soccer scenarios. Their training process consisted of two stages.
Initially, they trained the robots in two key skills: getting back up after a fall and scoring against an opponent. Then, they trained the robots for full-fledged one-on-one soccer matches through self-play, with the opponent being generated from partially trained copies of the robots.
They utilized rewards, randomization, and perturbations to encourage exploration and ensure safe performance in real-world settings. The resulting agent shows impressive and flexible movement skills, such as rapid recovery from falls, walking, turning, and kicking, and it can effortlessly switch between these actions.
Following simulation training, the agent smoothly transitioned to real-world robots. This successful transfer was facilitated by a combination of targeted dynamic randomization, perturbations during training, and high-frequency control.
In experimental matches, robots trained with deep reinforcement learning improved significantly over baseline skills programmed through scripts. They walked 181 percent faster, turned 302 percent faster, kicked the ball 34 percent faster, and recovered 63 percent more quickly from falls.
Researchers also observed spontaneous behaviors like spinning and pivoting, which are difficult to program manually. These findings suggest deep reinforcement learning can effectively teach fundamental movements to humanoid robots, paving the way for more complex behaviors in dynamic environments.
Future research may explore training teams of multiple agents, although initial experiments showed less agility despite a division of labor. Researchers also aim to train agents solely based on onboard sensors, presenting challenges in interpreting egocentric camera observations without external data.
Related Article : OpenAI Invests Big on AI-Powered Humanoid Robots
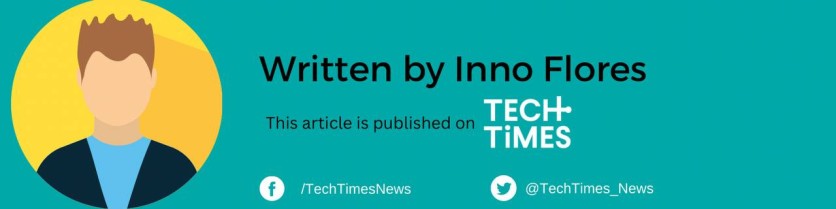
ⓒ 2025 TECHTIMES.com All rights reserved. Do not reproduce without permission.