The Allen Institute for AI (AI2) has unveiled an expansive open dataset named "Dolma," signaling a significant step towards the development of an open language model called OLMo.
The institute's intentions align with the principles of transparency and accessibility, aiming to provide the AI research community with both a comprehensible language model and an accessible dataset.
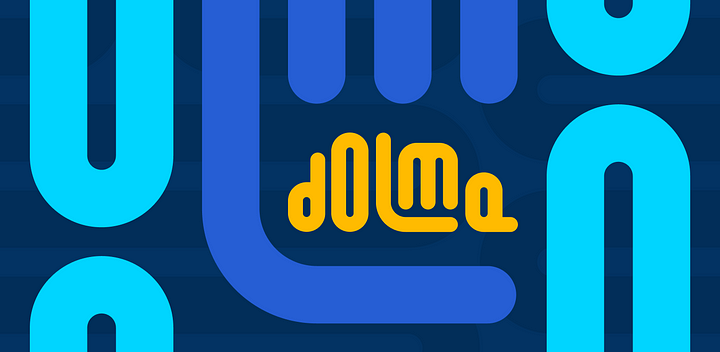
Dolma of AI2
The OLMo project, initiated in March, aims to foster the advancement of large-scale natural language processing (NLP) systems. A pivotal aspect of the project is the creation of OLMo using an open and transparent approach, supported by the release of pertinent artifacts and documentation detailing the project's progression.
AI2's recent release of the first data artifact in the initiative, Dolma, signifies a significant stride. Dolma encompasses an immense compilation of 3 trillion tokens sourced from an eclectic blend of content, including web resources, scholarly publications, code, books, and encyclopedic materials.
Notably, it emerges as the most substantial open dataset to date. The paramount considerations that guided the creation of Dolma are outlined in a comprehensive blog post by AI2. These considerations emphasize core principles such as openness, representativeness, size, reproducibility, and risk mitigation.
AI2 Creates Dolma
The creation of the Dolma dataset involved a meticulous and comprehensive process that transformed raw data from various sources into a coherent and cleaned dataset suitable for language model pretraining.
This process consisted of two primary categories of data processing: source-specific and source-agnostic operations. The first is source-specific operations. Each data source utilized in creating Dolma required unique processing to address its particular characteristics.
For instance, filtering files based on their software license was an operation exclusive to code sources. The process aimed to refine and structure the data while preserving its integrity.
The second category is source-agnostic operations. These were applied across multiple data sources and aimed to standardize the dataset. For example, removing personally identifiable information (PII) or decontaminating against an evaluation set were common source-agnostic operations.
These steps ensured the dataset adhered to a consistent structure and met ethical and privacy standards. Creating Dolma necessitated a combination of both types of operations, with multiple transformations executed in a pipeline fashion.
Some of the specifics involved in the process include handling web data from a common crawl where the web data underwent several rounds of deduplication to maintain data integrity.
Additionally, specific language filters tailored for web text were applied to enhance textual content quality. Code data also underwent a specialized cleansing process. Due to the distinct nature of code, certain preprocessing steps unique to code sources were applied to enhance its usability.
"Dolma differentiates itself from other datasets on two key aspects. First, it is significantly larger than other open datasets. Second, it is released under AI2's impact license, which was designed to balance ease of access with mitigation of potential risk in distributing large datasets," the blog post reads.
Related Article : Can AI Bots Solve CAPTCHA Tests Faster and More Accurately Than Humans? Researchers Reveal the Surprising Answer
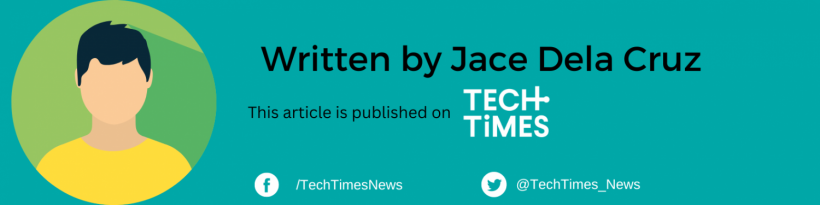
ⓒ 2025 TECHTIMES.com All rights reserved. Do not reproduce without permission.