Apple researchers have unveiled a potential solution to a significant challenge faced by large language models - the limitation of device memory.
With powerful models requiring substantial memory for storage, conventional smartphones, such as the iPhone 15 with 8GB of memory, struggle to meet the demands of models with potentially hundreds of billions of parameters.
The tech giant has unveiled a method designed to address this memory constraint. The approach involves efficient data transfers between flash memory and dynamic random-access memory (DRAM), enabling the execution of robust AI systems on smart devices, Tech Xplore reported.
"Our method involves constructing an inference cost model that harmonizes with the flash memory behavior, guiding us to optimize in two critical areas: reducing the volume of data transferred from flash and reading data in larger, more contiguous chunks," the researchers wrote in their paper.
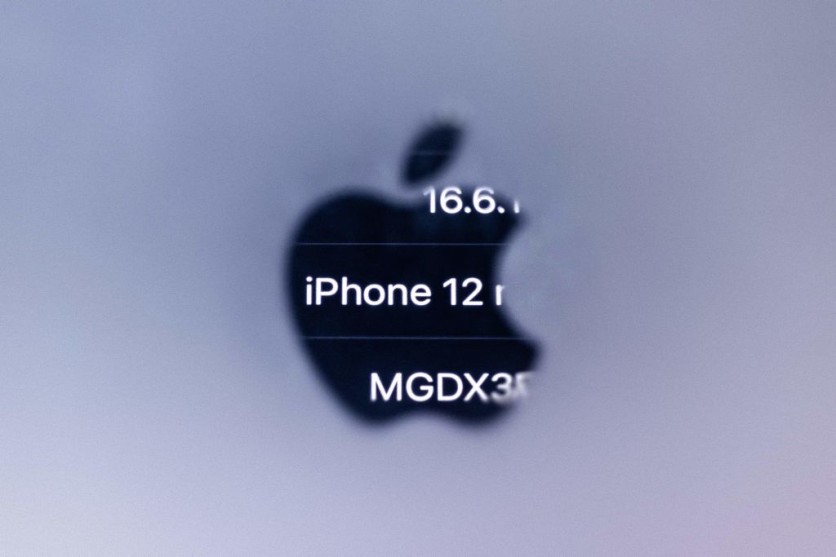
Twice the Size of DRAM
According to the researchers, their method can handle AI programs twice the size of a device's DRAM capacity, leading to a potential 500% increase in CPU speed and up to a 25-fold acceleration in GPU processes compared to current methods.
The researchers described their approach as crucial for deploying advanced Large Language Models (LLMs) in resource-limited environments, expanding their applicability and accessibility.
Their method employs two key techniques: windowing and row-column bundling. Windowing reduces the volume of data exchanged between flash memory and RAM by reusing recent calculation results, minimizing input-output requests, and saving energy and time.
Row column bundling enhances efficiency by processing larger data chunks at once from flash memory. The researchers noted that these techniques contribute to significantly reducing data load and enhancing memory usage efficiency. This breakthrough, they said, is especially vital for deploying advanced LLMs in environments with limited resources.
Beyond Memory Constraints
The potential impact of this development extends beyond memory constraints. With the growing capabilities of smart devices, enhancing their performance could lead to more sophisticated interactions.
According to the research team, from in-depth natural language exchanges to checking vital signs through a global database, providing real-time translation, and even creating animated avatars from single-lens videos, these advancements signal a trajectory toward more powerful and versatile AI capabilities integrated into our daily lives.
Apple has been making strides in various AI-related areas, such as the recent announcement of a program called HUGS, which can create animated avatars from short video clips captured from a single lens, offering a faster and more efficient approach than existing methods.
"These methods collectively enable running models up to twice the size of the available DRAM, with a 4-5x and 20-25x increase in inference speed compared to naive loading approaches in CPU and GPU, respectively," the paper's abstract reads.
"Our integration of sparsity awareness, context-adaptive loading, and a hardware-oriented design paves the way for effective inference of LLMs on devices with limited memory," it added.
The study of the Apple research team, titled "LLM in a Flash: Efficient Large Language Model Inference with Limited Memory," was recently published in the pre-print server arXiv.
Related Article : Apple AI Training: Looking for $50 Million Worth of Licensed News for its Data Needs
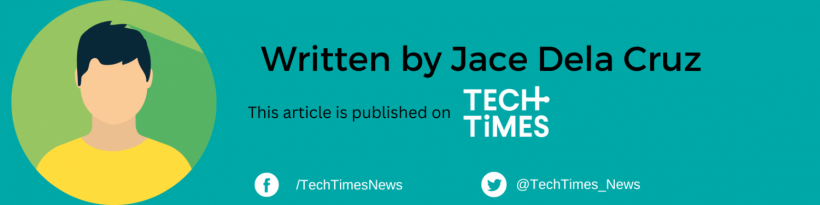
ⓒ 2025 TECHTIMES.com All rights reserved. Do not reproduce without permission.