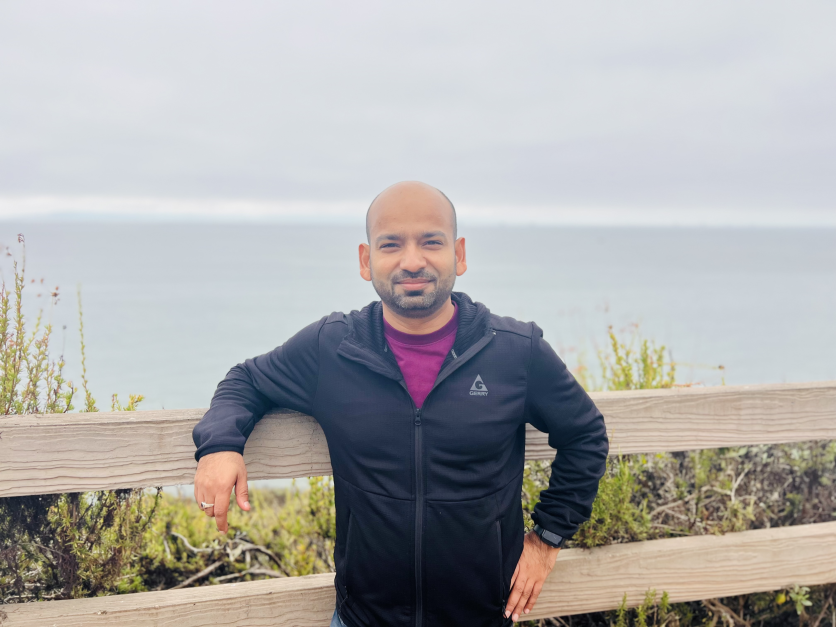
In an era when data fuels innovation and AI defines competitive advantage, large-scale cloud data platform modernization is at the forefront of enterprise strategy. He is a Senior Engineering Manager with 19+ years in data engineering, known for leading transformations across Amazon Web Services (AWS), Google Cloud Platform (GCP), and Databricks.
Shishir Tewari's work spans building massive data ecosystems and integrating AI/ML to optimize them. Notably, he managed Google's Finance Data Universe, handling over 100 petabytes, spearheaded a Snowflake-to-Databricks migration, and built AWS-based data lakes—all endeavors that dramatically improved efficiency and analytics capabilities.
These achievements underscore his reputation for driving AI/ML-powered optimization of enterprise data platforms. Currently, Tewari leads the development of a next-generation MDM platform at Procore Technologies, bringing AI/ML into core data processes to boost accuracy, governance, and real-time insight.
His career includes leadership roles at Google, Amazon, Morgan Stanley, and J.P. Morgan, where he modernized data infrastructure and promoted AI-driven analytics. This broad experience in cloud data engineering and business intelligence has cemented Tewari as a thought leader in applying AI/ML at scale.
In 2024, he authored a book titled "AI-Driven Enterprise: Scaling Business Success," now available on Amazon. This publication reflects Tewari's belief that high-quality data is central to successful AI strategies and further demonstrates his commitment to advancing AI-driven data practices.
Enterprises are increasingly shifting from traditional data warehouses to flexible "lakehouse" architectures for analytics, seeking cost efficiency and scalability. At the same time, artificial intelligence is being applied to automate data management tasks, improving data quality and accelerating insights.
Tewari's work sits at this intersection of cloud and AI—modernizing data platforms while infusing AI to unlock their full potential.
AI-Powered Master Data Management at Procore
"As a developing company, we depend on effective master data management," Tewari says of his current work at Procore. "At Procore, we included AI/ML in our MDM processes to automate data classification, validation, and enrichment, which set us apart."
Integrating machine learning into MDM has allowed his team to systematically organize and clean core business data, a task that is traditionally labor-intensive. This approach exemplifies a wider industry trend: companies are increasingly turning to AI to enhance data validation and enrichment for greater efficiency and accuracy.
By letting algorithms handle the grunt work of data cataloging and quality control, Procore's data platform can scale without compromising integrity. Tewari is spearheading Procore's next-gen MDM platform with a clear goal—"enhance data accuracy, governance, and real-time insights" through AI/ML.
In practice, this means using intelligent models to deduplicate records, enforce data standards, and deliver up-to-date information for decision-makers. Such AI-powered MDM strategies help modern businesses make well-informed choices by ensuring a single source of truth.
Tewari's leadership in this area is driving higher confidence in Procore's data. It also illustrates how embracing AI in data management can yield cleaner, more trustworthy data assets, which in turn power more reliable analytics and operations.
Migrating from Snowflake to Databricks for Cost-Efficient Analytics
In a recent initiative at Procore, Tewari led a major cloud modernization project: "I led the migration from Snowflake to Databricks, optimizing cloud performance and reducing costs." Moving a large-scale data warehouse between platforms is no small feat, but it paid off by lowering latency and consolidating data workflows.
Many organizations are making similar shifts toward open lakehouse architectures—in fact, 65% of enterprises now run the majority of analytics on data lakehouses, citing cost efficiency and ease of use as top reasons. By adopting Databricks, which unifies data warehousing with AI-friendly data lakes, Tewari aligned Procore's architecture with this industry momentum toward more flexible, economical analytics platforms.
"This migration was key to enabling advanced AI-driven analytics across the company," he adds, highlighting the strategic upside beyond cost savings. On Databricks, Procore's data teams can tap into native ML capabilities and process large datasets more seamlessly for machine learning.
The benefits mirror those reported elsewhere—for example, one company cut total data infrastructure costs by 76% after switching from Snowflake to a Databricks lakehouse. Tewari's experience demonstrates how upgrading cloud data infrastructure can both save money and open the door to deeper AI and analytics.
By unshackling data from a closed warehouse and turning it into a versatile lakehouse, Procore improved its performance and gained the freedom to innovate with AI on its data.
Managing a 100+ PB Finance Data Universe at Google
Tewari's tenure at Google showcased his ability to handle data on an extreme scale. "At Google, I managed the Google Finance Data Universe, processing over 100 petabytes of financial data," he recalls.
This gargantuan platform aggregated global revenue and finance data, demanding a highly scalable architecture. Handling data at such a magnitude is a monumental task—Uber's Hadoop-based big data platform, for instance, manages over 100 petabytes of analytical data while maintaining minute-level query latencies.
Tewari's work was similar in scope. By leveraging Google's internal technologies (BigQuery, MapReduce, etc.), his team was able to churn through massive datasets and still deliver timely insights for the business.
Leading this finance data engineering effort also meant coordinating a large, distributed team. Tewari notes that he "led a global team of over 15 data engineers" to maintain and improve the Finance Data Universe.
Under his leadership, the team not only wrangled data volume but also improved Google's revenue allocation efficiency by refining how financial data was processed and reported. It's a prime example of how big data, when properly managed, can directly impact business outcomes.
Google's own engineering culture provided the tools and scale—for context, Google was running 100,000+ MapReduce jobs daily as far back as 2008, processing 20 petabytes per day. Building on that foundation, Tewari's global team enhanced the finance data platform's performance, ensuring that revenue streams were accurately tracked and allocated at a planetary scale.
Building AWS Big Data Infrastructure for Amazon Advertising
Transitioning to Amazon, Tewari applied his data prowess to the realm of digital advertising. "At Amazon, I built an advertising big data infrastructure on AWS," he says, describing a cloud-native data lake that captured and analyzed advertising metrics in real-time.
This infrastructure ingested streams of ad impressions, clicks, and conversions, and stored them in scalable AWS data lakes for analysis. The stakes were high: Amazon's advertising arm has grown into a $56+ billion business as of 2024, making it the third-largest digital advertiser.
To support that level of revenue, Amazon's ad platform must process a firehose of user and marketplace data. Tewari's solution leveraged AWS services (like Kinesis for streaming and S3 data lakes) to handle the volume and velocity of ad data with ease.
Critically, Tewari engineered the system with efficiency in mind—"optimizing costs and improving real-time analytics capabilities," as he puts it. By fine-tuning data pipelines and storage choices, he helped cut unnecessary cloud expenditures while speeding up data availability for stakeholders.
Real-time insights are especially vital in advertising, where delays can mean missed opportunities to adjust campaigns. Modern cloud architectures enable this: AWS offers reference models for real-time advertising analytics that emphasize low-latency data processing.
In Tewari's case, his optimized AWS data lake allowed Amazon's marketing teams to query fresh data on the fly and react to customer behavior instantly. Moreover, cost optimizations ensured these powerful capabilities remained economically sustainable at Amazon's massive scale.
Tewari's work on AWS exemplified how to balance scale, speed, and cost—a trifecta for any big data system in the advertising domain.
Driving Data Compliance and Risk Analytics at Morgan Stanley
Before his tech industry roles, Tewari made a significant impact in finance by modernizing data for regulatory compliance. "At Morgan Stanley, I led data engineering teams responsible for regulatory compliance and risk analytics," he explains.
In a global bank, these functions generate enormous data needs—from trade records for compliance to market data for risk models. Tewari's teams developed data pipelines to aggregate and prepare this information for analysis and reporting.
Notably, he worked on high-stakes risk management projects, and he notes this included crucial "liquidity stress testing" processes for the bank. Such stress tests simulate crisis scenarios and require crunching vast amounts of financial data to ensure the bank meets Basel III regulatory standards.
(Basel III is an international regulation that requires banks to use quantitative data models for risk projection and to report routinely the results across the organization.) Tewari's efforts at Morgan Stanley coincided with the firm's broader push to overhaul data quality and governance.
The bank had recognized that if data feeding risk or compliance systems are inaccurate, it could lead to flawed decisions—an unacceptable outcome. As a result, Morgan Stanley established a centralized data center of excellence in 2018 to improve core data quality and consistency across all divisions.
Within this environment, Tewari's team delivered unified, trustworthy datasets for regulatory reporting and risk analytics. By automating data integration and validation for compliance, they reduced manual errors and ensured timely reporting to regulators.
In the highly regulated world of finance, Tewari's work demonstrated how modern data engineering (with strong governance) helps institutions not only meet compliance requirements but also glean analytical insights (like risk forecasts) that inform strategy. It was a balancing act of innovation and caution—one that he navigated adeptly through robust data architecture and governance practices.
Streamlining Critical Data Pipelines at J.P. Morgan
At J.P. Morgan, Tewari continued his mission of making data infrastructure more efficient and reliable. "At J.P. Morgan, I optimized ETL pipelines and SQL models," he says, referring to the extract-transform-load workflows and database logic that underlie the bank's analytics and reporting.
These optimizations were not merely technical tweaks but essential upgrades in a data ecosystem of immense scale—J.P. Morgan Chase's data infrastructure includes over 450 petabytes of data serving more than 6,500 applications. In such an environment, streamlining data pipelines can have a huge payoff.
Tewari redesigned data workflows to eliminate bottlenecks and redundancies, ensuring that data moved smoothly from source systems (trading platforms, ledgers, etc.) into data warehouses and analytic models. Crucially, Tewari also overhauled "financial data processes for critical reporting systems," meaning he improved how key business reports (e.g., regulatory filings, profit/loss reports, risk reports) are generated from raw data.
By refining these processes, he cut down the time analysts spent wrangling data and increased the trustworthiness of the outputs. This addresses a common pain point: it's estimated that most data professionals spend only 20% of their time on actual analysis and 80% on gathering and cleaning data.
Tewari's pipeline optimizations reversed that ratio for J.P. Morgan's critical reports—automating data prep and quality checks so that finance teams could focus on analysis rather than janitorial work. The outcome was faster, more accurate reporting to executives and regulators.
In a bank that processes billions of data points daily, these efficiencies were a game changer. Tewari's work at J.P. Morgan highlights how meticulous data engineering can reduce operational drag and enable a data-driven organization to act with speed and confidence.
Multi-cloud Expertise and Data Governance Frameworks
Throughout his career, Tewari has developed a broad and deep technical skillset that underpins his success in data engineering. "I have deep experience in Python, SQL, Spark, and cloud platforms like AWS, GCP, and Databricks," he notes, reflecting a versatility across programming, big data processing, and multi-cloud environments.
This expertise allowed him to choose the right tool for each job—whether it was using Spark for distributed data transformations or leveraging a specific cloud service to optimize performance. Tewari's proficiency across AWS, Google Cloud, and Databricks also meant he could lead teams in hybrid cloud settings and integrate diverse technologies.
Many enterprises today adopt a multi-cloud strategy to avoid vendor lock-in and to use the best services from each provider. Engineers like Tewari, fluent in all major cloud stacks, are thus extremely valuable in designing interoperable data solutions.
Beyond tools and platforms, Tewari emphasizes the importance of strong foundations in any data initiative. He is a strong proponent of "data governance frameworks"—formal processes and policies to manage data quality, security, and lifecycle.
In his view, having robust data governance is critical before layering on advanced AI analytics. Industry experts agree: effective data governance forms the foundation for AI systems that enhance strategic decision-making and mitigate risks.
In practice, Tewari has implemented governance measures like standardized data definitions, access controls, and quality monitoring in the platforms he built. This ensures that data is reliable and compliant, which in turn makes AI/ML results trustworthy.
By coupling technical prowess with governance discipline, Tewari creates data environments where innovation can thrive without sacrificing integrity. It's a balanced approach that yields scalable, sustainable data platforms—a hallmark of his technical leadership.
Thought Leadership in AI and the Future of Data Engineering
Beyond his core responsibilities, Tewari makes it a priority to share his expertise with the broader data engineering community. In his 2024 book, AI-Driven Enterprise: Scaling Business Success, he emphasizes that even the most sophisticated AI models are only as good as the data they rely on. By outlining best practices for data cleaning, integration, and governance, Tewari offers a roadmap for organizations seeking to unlock meaningful results from generative AI and machine learning initiatives.
In addition to writing, Tewari stays active in the professional community. He has authored multiple scholarly articles on big data and AI, and he often serves as a judge or panelist in industry data science competitions and innovation awards.
These roles allow him to mentor emerging talent and evaluate cutting-edge projects at the intersection of data and AI. "I have a passion for technical strategy, innovation, and mentoring future leaders," Tewari says, expressing why he devotes time to these activities.
His involvement in judging panels—for example, the Global AI Recognition Awards—showcases his expertise in assessing how well others harness data solutions at scale. By sharing knowledge and recognizing excellence, Tewari contributes to advancing the field as a whole.
It's clear that he views leadership as more than delivering on his projects; it's also about uplifting the data engineering discipline. This combination of practical experience and forward-thinking vision positions Tewari as a key voice in how AI-driven data engineering will evolve in the years ahead.
From modernizing legacy data pipelines to injecting AI into cloud platforms, Tewari's journey illustrates how purposeful data engineering can transform enterprises. He has consistently bridged the gap between massive data and meaningful insight—whether by cutting costs with a new lakehouse, accelerating compliance reporting, or pioneering AI-enhanced data management.
Tewari's story is a testament to the power of combining technical expertise with strategic vision and governance. As businesses everywhere race to become more data-driven and AI-powered, leaders like Tewari provide a blueprint for success: invest in quality data foundations, embrace innovative technologies judiciously, and never lose sight of the business impact.
With his ongoing contributions and mentorship, Tewari continues to shape the future of data engineering, proving that with the right strategy, data and AI can unlock extraordinary value across industries.
ⓒ 2025 TECHTIMES.com All rights reserved. Do not reproduce without permission.