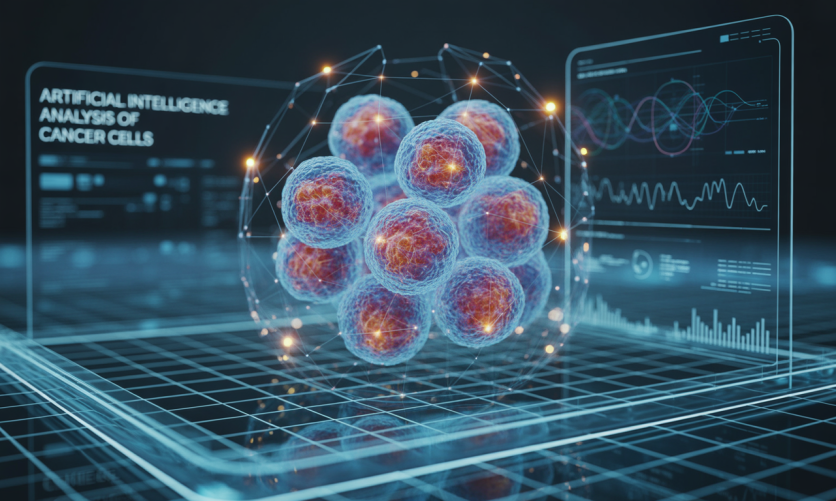
The intersection of artificial intelligence (AI) and healthcare presents a transformative frontier, particularly in the global fight against cancer. As healthcare systems worldwide grapple with rising cancer rates—with nearly 20 million new cases diagnosed globally in 2022 and projections suggesting a 77% increase by 2050—and the complexities of diagnosis and treatment, AI-driven tools offer the potential to enhance efficiency, improve accuracy, and personalize care.
The global AI in oncology market reflects this potential, valued between approximately $1.2 billion and $1.92 billion in 2023. It is projected to grow significantly, potentially reaching over $9 billion to $11.5 billion by 2030 or 2031.
However, the deployment of these sophisticated technologies, especially in emerging markets facing unique resource constraints and infrastructure challenges, necessitates a careful evaluation of their costs and benefits. Understanding the practical implications, ethical considerations, and future trajectory of AI in oncology within these specific contexts is crucial for ensuring equitable access and sustainable healthcare solutions.
Guru Lakshmi Priyanka Bodagala, a Health Informatics Analyst and Digital Health Specialist based in San Francisco, California, brings a unique perspective to this critical discussion. With a Doctor of Pharmacy degree from Rajiv Gandhi University and a Master of Science in Digital Health Informatics from the University of San Francisco, she combines deep clinical knowledge with advanced technical expertise.
Her work focuses on leveraging real-world healthcare datasets—including Electronic Health Records (EHR), FHIR, and claims data—using machine learning, statistical modeling, and bioinformatics tools. These tools include BLAST (Basic Local Alignment Search Tool) and HMMER (which utilizes profile Hidden Markov Models) to extract actionable insights.
Bodagala's technical proficiency spans Python, SQL, PySpark, Snowflake, and deep learning frameworks like Keras, complemented by a strong understanding of medical terminologies and interoperability standards such as SNOMED, ICD-10, HL7, and FHIR.
Through internships at Motive Medical Intelligence and Modality.ai, Bodagala gained hands-on experience in FHIR data transformation, digital biomarker validation (objective measures collected via digital devices), and developing AI-based healthcare solutions. Her background encompasses clinical research, medical writing, pharmacotherapy, and toxicology.
Currently, her passion lies in harnessing data and AI to revolutionize healthcare delivery, with a specific focus on genomics-driven cancer prediction models, EHR-based Natural Language Processing (NLP) for disease classification, and the ethical deployment of generative AI for clinical decision support. Bodagala's insights on the cost-benefit dynamics of AI cancer prediction models in resource-limited settings reveal the critical factors at play, drawing upon her specialized knowledge in health informatics, bioinformatics, clinical research, AI in healthcare, digital biomarkers, EHR NLP, FHIR integration, genomics, and clinical decision support.
The Pivotal Moment: AI's Potential in Underserved Settings
The potential for AI to revolutionize cancer care in resource-limited environments often stems from recognizing the profound impact of diagnostic delays. Analyzing healthcare data reveals stark correlations between late diagnoses, increased treatment expenditures, and poorer patient outcomes, particularly in underserved populations where cancer mortality rates can be significantly higher despite lower incidence compared to high-income countries.
Bodagala recalls a specific point where this connection became undeniable through her work. "The realization struck during my work analyzing cancer prevalence in underserved populations using publicly available health datasets," she explains. "I noticed how delays in diagnosis often resulted in higher treatment costs and worse outcomes." This observation highlighted a critical gap where technology could potentially intervene, as early cancer detection significantly improves survival and lowers treatment costs.
The true turning point came when applying early AI models to this problem. By utilizing minimal clinical and demographic data readily available even in low-resource settings, the possibility of identifying high-risk individuals emerged.
This predictive capability suggested a pathway to scalable, cost-effective interventions that could mitigate the consequences of delayed diagnosis, a crucial need given that cancer cases and deaths are projected to increase dramatically in low-HDI countries by 2050. "When I ran early AI models to predict high-risk individuals using minimal clinical and demographic data, the potential was clear: timely, cost-effective intervention could be scaled even in low-resource settings," Bodagala states.
"That was a pivotal moment—I saw AI not just as a research tool but as a practical force for equity in care," aligning with the potential for AI to reduce burdens on strained healthcare facilities.
Bridging Clinical Insight and Technical Evaluation
Evaluating AI models for healthcare, especially in emerging markets, requires more than just assessing technical accuracy; it demands a deep understanding of the clinical context. Bodagala emphasizes that her background in pharmacotherapy and toxicology provides a crucial lens for this evaluation, ensuring that AI outputs are not only statistically sound but also practically applicable and safe within specific regional constraints.
"My clinical background grounds my technical evaluation in real-world applicability," she notes. "Understanding drug mechanisms and patient variability helps me assess whether AI predictions are not only accurate but also actionable." This aligns with the broader need for AI systems to move beyond pattern recognition to offer clinically relevant insights.
This dual perspective allows for a more holistic assessment of an AI model's value, moving towards the goal of precision medicine tailored to individual characteristics. For instance, identifying a high-risk patient is only the first step; the clinical feasibility of subsequent actions within the local healthcare system is paramount.
Bodagala elaborates, "For example, if a model flags a high-risk patient, I can evaluate which therapies are available, cost-effective, and safe in that particular region." This integration of clinical knowledge ensures that the AI tools developed are fit for purpose, addressing concerns that AI recommendations must be validated within the specific clinical scenario.
"This dual lens ensures our AI tools are not just scientifically sound but also ethically and clinically relevant in emerging healthcare systems," she concludes, highlighting the importance of context-specific validation.
Key Metrics in Cost-Benefit Analysis for AI Cancer Tools
Conducting a thorough cost-benefit analysis for AI-driven cancer prediction tools involves looking beyond simple accuracy metrics. Bodagala identifies a multifaceted approach focusing on diagnostic performance, economic impact, and system-level efficiencies.
"I typically focus on three pillars: diagnostic accuracy (AUC, sensitivity, specificity), intervention cost reduction (earlier-stage treatment expenses vs. late-stage), and system-level impact (reduced hospitalizations, optimized provider time)," she outlines. These quantitative measures provide a baseline for evaluating the tool's direct contributions, reflecting the understanding that AI can improve the cost-benefit ratio of treatment by enabling earlier detection and potentially reducing unnecessary procedures.
However, the assessment extends to qualitative factors crucial for real-world adoption, particularly the interpretability of the AI's output. If clinicians cannot understand or trust the predictions—a common concern hindering AI adoption—the potential benefits, including cost savings, may never be realized.
Bodagala adds, "Additionally, I evaluate model interpretability—because if clinicians can't understand or trust the output, the cost savings won't materialize." Ultimately, the ROI encompasses more than just financial gains; it includes broader healthcare improvements.
"ROI is not just financial; it includes improved patient outcomes, better care access, and staff efficiency," she asserts, emphasizing a holistic view of value that aligns with AI's potential to optimize healthcare workflows and reduce clinician workload.
Leveraging Interpretability for Stakeholder Trust
While direct engagement with stakeholders in under-resourced environments is a future goal, Bodagala highlights the proactive use of interpretability techniques like SHAP (Shapley Additive Explanations) during model development as crucial for building trust and facilitating future adoption. Transparency is key in any clinical setting, and SHAP provides a mechanism to understand why an AI model makes a specific prediction by assigning contribution values to each feature.
"While I haven't yet worked directly with healthcare stakeholders in under-resourced environments, I've used SHAP during model development to ensure interpretability and transparency—critical components for clinical adoption in any setting," she explains, addressing the "black-box" problem often cited as a barrier.
Providing concrete examples, Bodagala illustrates how SHAP clarifies model reasoning in her cancer prediction projects, similar to how it has been used in other oncology studies to delineate feature importance for prognostication or diagnosis. "For instance, in one of my cancer prediction projects using genomic and clinical data, SHAP helped identify which features (such as age, tumor stage, or mutation patterns) were most influential in survival prediction."
This level of detail, explaining feature influence on outcomes like tumor size or texture, is invaluable, especially where resources are limited. "These insights can be invaluable for future deployment in low-resource areas where clinical expertise or diagnostic tools may be limited, as they provide a clear rationale behind each prediction, enhancing trust and usability," she notes, underscoring AI decision-making with SHAP and trustworthiness for clinicians.
Balancing Financial Constraints and Long-Term Benefits
Designing AI models for early cancer detection in settings with immediate financial constraints requires a strategic approach that demonstrates value quickly while building a case for sustained investment. High initial costs, including data acquisition potentially exceeding $1 million annually, are significant barriers.
Bodagala focuses on solutions that minimize initial costs and leverage existing infrastructure. "We prioritize scalable solutions that require minimal upfront investment—such as using existing EHR data, lightweight algorithms, or cloud-based platforms," she says. The key is to show tangible short-term gains that resonate with budget-conscious decision-makers.
Demonstrating immediate impact helps bridge the gap between upfront costs and long-term advantages. "By demonstrating quick wins, like reduced emergency visits or shorter diagnostic timelines, we build the case for long-term investment," Bodagala explains.
This involves not just technological design but also collaborative efforts to shift perspectives on preventative care, aligning with the idea that AI efficiencies can improve cost-benefit ratios and allow saved costs to be reallocated. "I also collaborate with interdisciplinary teams to develop value-based care models that reframe early detection as a cost-saving initiative, not a luxury," she adds, highlighting the need for both technical and systemic approaches to overcome financial barriers.
Navigating Data Integration Challenges in Cost-Effective Solutions
Integrating complex datasets, such as those involving FHIR standards and digital biomarkers, into cost-effective AI solutions presents significant hurdles, particularly concerning data quality and consistency. While standards like FHIR aim to improve interoperability by defining how healthcare information can be exchanged between systems, practical implementation reveals ongoing challenges with data fragmentation.
Bodagala points out, "The main challenge is data heterogeneity—FHIR standards help, but data quality and completeness still vary widely." This variability is often more pronounced in resource-limited settings.
Addressing these data challenges requires sophisticated preprocessing and adaptable modeling techniques. "In resource-limited settings, we often deal with partial records, missing biomarker data, or paper-based inputs," Bodagala notes. "We address this with preprocessing pipelines, hybrid data ingestion models, and imputation techniques that maintain model performance while minimizing infrastructure demands."
Successfully merging diverse data types, including integrating multi-omics data like genomics, transcriptomics, and metabolomics, which are increasingly used for biomarker discovery, is fundamental to creating impactful AI tools. "Aligning clinical, genomic, and operational data is tough—but essential for truly impactful AI," she concludes, emphasizing the critical nature of robust data integration strategies.
Adapting Privacy and Regulatory Compliance Across Infrastructures
Deploying AI systems across diverse healthcare environments necessitates a flexible yet rigorous approach to regulatory compliance and data privacy, adapting to varying levels of digital infrastructure while upholding core principles. Bodagala stresses that adherence to standards like HIPAA and protecting Protected Health Information (PHI) is fundamental, but the methods must suit the context.
"Regulatory compliance is non-negotiable, but it requires flexibility," she states. "In high-resource settings, we follow full HIPAA and PHI protocols; in emerging markets, we design privacy-first architectures using federated learning or edge computing, so data never leaves the source." Federated learning, a technique where models are trained decentrally without sharing raw patient data, is gaining traction as a key privacy-enhancing technology.
This commitment to privacy extends beyond mere compliance, embedding security measures like encryption and access controls deeply within the AI system's design, regardless of the environment's technological maturity. "Transparency, encryption, and audit trails are baked into every layer of model deployment," Bodagala explains.
The overarching goal is to ensure ethical AI use universally, addressing major concerns about data misuse and security breaches. "Our approach is to elevate privacy protections regardless of infrastructure maturity, ensuring ethical AI use across environments," she affirms, highlighting a principled stance on data protection.
Emerging Trends for Sustainable AI in Underserved Communities
Looking ahead, Bodagala envisions the future of AI-driven cancer prediction in underserved communities centering on decentralized, interpretable, and privacy-preserving technologies that empower local healthcare systems. "The future lies in decentralized, interpretable AI models powered by privacy-preserving technologies," she predicts, echoing the potential of federated learning to enable collaborative AI without compromising privacy.
This involves shifting care closer to the patient through innovative screening and triage methods. "Community-based screening with mobile-enabled diagnostics, combined with AI triage tools, will shift the point of care closer to patients," potentially leveraging AI to overcome geographical barriers and personnel shortages.
Sustainability also hinges on developing AI models trained on diverse, real-world data to ensure they are inclusive and effective across different populations, mitigating risks of algorithmic bias stemming from unrepresentative datasets. "We're also seeing growth in AI/ML models trained on diverse, real-world datasets—making them more inclusive and generalizable," Bodagala observes.
The ultimate aim is to create AI systems that integrate seamlessly into existing workflows and strengthen local healthcare capacity. "Ultimately, sustainability will come from AI systems that empower local healthcare providers, reduce dependency on centralized labs, and integrate seamlessly into existing workflows," she concludes, outlining a vision for AI as an enabler of self-sufficient and equitable healthcare.
Bodagala's insights illuminate the complex interplay between technological innovation, clinical applicability, economic realities, and ethical imperatives in deploying AI for cancer prediction, particularly within emerging markets. Her perspective underscores that realizing AI's potential—reflected in a rapidly growing global market—requires not only sophisticated algorithms but also a deep understanding of local contexts, a commitment to data privacy and interpretability through techniques like federated learning and privacy-preserving AI techniques, and strategic approaches to demonstrate value amidst financial constraints.
The path forward involves prioritizing scalable, cost-effective solutions, fostering interdisciplinary collaboration, navigating data integration challenges, and ensuring that AI tools empower local healthcare providers, ultimately aiming to bridge global health disparities and create sustainable, equitable cancer care solutions for underserved communities worldwide.
ⓒ 2025 TECHTIMES.com All rights reserved. Do not reproduce without permission.