A team of researchers from Carnegie Mellon University has developed a new robotic agent named ALAN, which is capable of autonomously exploring unfamiliar environments, according to a report by TechXplore.
Russell Mendonca, one of the researchers involved in the study, explained that the team was interested in creating an AI that could learn by setting its own objectives.
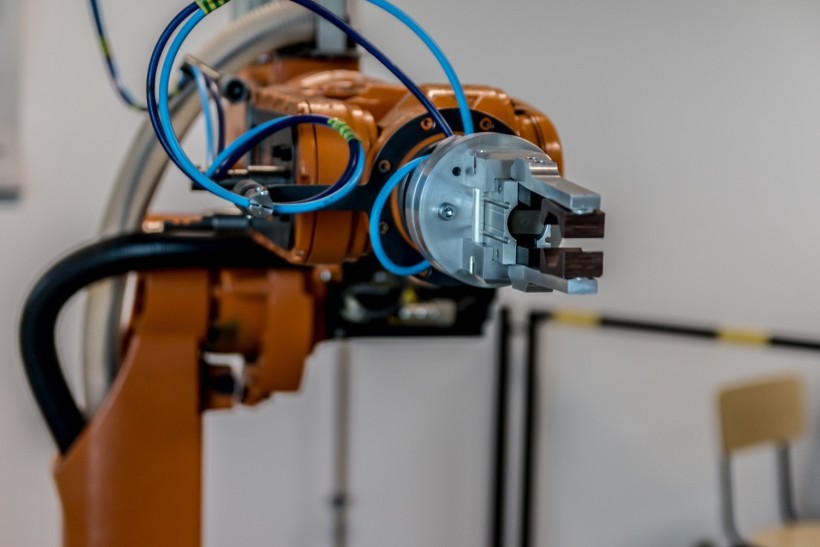
By eliminating the need for human supervision or guidance, such agents can continue to learn and adapt to new scenarios, driven by their own curiosity. This approach would enable agents to generalize across different domains and discover increasingly complex behaviors.
While the robotics group at Carnegie Mellon University had previously introduced autonomous agents that could perform well on new tasks with minimal additional training, these models were only tested in simulated environments.
Environment- and Agent-centric Objectives
According to the research team, ALAN learns a world model to plan its actions and uses environment-centric and agent-centric objectives to direct itself.
Additionally, it reduces the workspace to the area of interest using off-the-shelf pre-trained detectors. After exploration, the robot can stitch the discovered skills to perform single and multi-stage tasks specified via goal images.
The robot developed by the team comes equipped with a visual module that can estimate the movements of objects in its surroundings.
This module then uses these estimations to encourage the robot to interact with these objects and maximize the change in them.
By developing ALAN, the team has made significant progress in creating an autonomous system that can explore its surroundings and complete new tasks without relying on human guidance or rewards.
According to Mendonca, the environment-centric signal that ALAN receives is not dependent on the agent's belief. To improve its estimate of the change in objects, ALAN needs to be curious and use its learned model of the world to identify actions where it is uncertain about the predicted object change.
It then executes these actions in the real world to improve its agent-centric signal, which evolves as the robot sees more data.
Read Also: Tiny Robot Mimics Geckos and Inchworms, Paves the Way for Human Surgical Applications
ALAN's Learning Approach
Unlike other proposed approaches for autonomous robot exploration, the learning approach developed by Mendonca and his colleagues allows the ALAN robot to continuously and autonomously learn new tasks without the need for large amounts of training data.
Mendonca stated that using visual priors can greatly increase the efficiency of robot learning, as demonstrated by ALAN's ability to learn how to manipulate objects with only around 100 trajectories in 1-2 hours in two different play kitchens, without any rewards.
The researchers are now interested in studying how to utilize other priors, such as videos of humans performing tasks and language descriptions, to help structure the robot's behavior and in multi-robot systems that can pool their experience to continually learn.
The framework they have created has the potential to revolutionize the way physical robots operate and interact with their environment.
The team's findings were published in arXiv.
Related Article: Engineers Create One of the Fastest, Most Efficient Amphibious Robots Inspired by Flippers and Centipedes