Web publishers now have a choice whether to allow their web content to be utilized by Google as material to feed its Google Bard and any future AI models it decides to make.
This choice can be exercised by implementing a simple measure: disallowing "User-Agent: Google-Extended" in the robots.txt file of their website, which dictates the content accessible to automated web crawlers.
This development empowers web publishers with greater control over how their content contributes to emerging generative AI applications.

Google-Extended
Google has launched Google-Extended, offering web publishers a tool to manage their sites' involvement in refining Bard and Vertex AI generative APIs, including forthcoming model iterations. This tool allows website administrators to make informed decisions about using their content to enhance AI capabilities.
"By using Google-Extended to control access to content on a site, a website administrator can choose whether to help these AI models become more accurate and capable over time," the VP of Trust, Danielle Romain, wrote in a blog post.
By incorporating Google-Extended into robots.txt, Google aims to provide a transparent and scalable control mechanism for web publishers. As AI applications continue to evolve, managing diverse uses on a large scale may pose challenges for web publishers.
In response, Google expressed commitment to collaborate with both the web and AI communities to explore additional machine-readable solutions for choice and control, with further updates anticipated in the near future.
"Making simple and scalable controls, like Google-Extended, available through robots.txt is an important step in providing transparency and control that we believe all providers of AI models should make available. However, as AI applications expand, web publishers will face the increasing complexity of managing different uses at scale," Romain said.
"That's why we're committed to engaging with the web and AI communities to explore additional machine-readable approaches to choice and control for web publishers. We look forward to sharing more soon," she added.
Read Also : Google's Bard AI Chatbot Can Now Talk in Over 40 Languages and Respond to Visual Prompts After Latest Update
How Models Learn From Data on the Web
Large language models learn from data on the web through a process called unsupervised learning. It begins with collecting a diverse range of text from sources like articles, websites, forums, and more.
The text is then broken down into smaller units called tokens, which could be as short as a character or as long as a word. This data undergoes preprocessing steps like converting to lowercase and removing punctuation.
The model aims to predict the next token in a sequence based on the ones that came before it. It looks at a context window of tokens to make these predictions.
The model is built using a deep neural network, allowing it to capture complex patterns. It goes through multiple training iterations, adjusting its internal parameters to minimize the difference between predicted and actual tokens.
Related Article : Google Focuses on 'Everyday' AI Features to Compete with ChatGPT and Bing: Generative AI Assistance
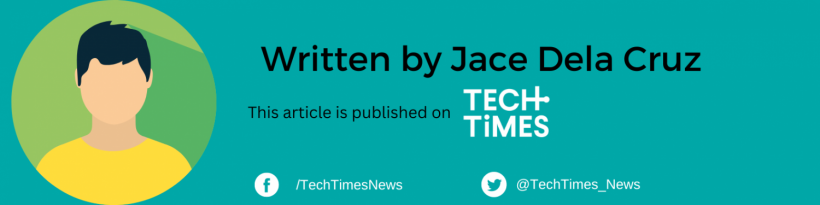
ⓒ 2025 TECHTIMES.com All rights reserved. Do not reproduce without permission.