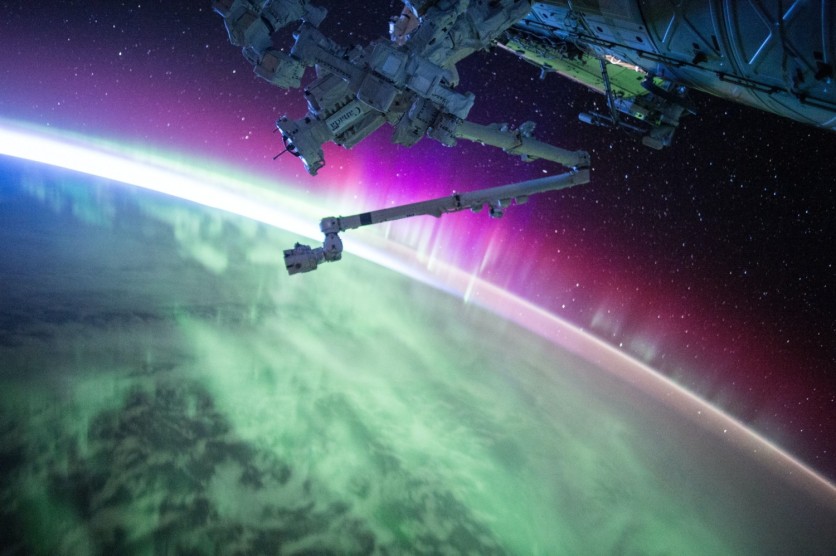
A worldwide network of researchers uncovered 11 previously unknown space anomalies, seven of which are supernova contenders, by using machine learning techniques to automate the search.
AI Discovers Space Anomalies
The majority of astronomical discoveries have been derived from studies followed by calculations. While the overall number of observations remained relatively low in the twentieth century, the volume of data significantly grew with the entrance of vast astronomical studies.
Manually analyzing such massive volumes of data is costly and time-consuming, so the SNAD team of academics from Russia, France, and the United States collaborated to create an automated solution.
The SNAD team is a consortium of researchers led by Matvey Kornilov, an Associate Professor of Physics at HSE University. These researchers recently published findings in the journal of New Astronomy, outlining how they use machine learning to help detect 11 previously unknown space anomalies. Seven of these are thought to be supernova candidates.
Using the' nearest neighbor' approach, the researchers used a k-D tree to discover abnormalities in digital photos of the Northern sky collected in 2018. In computer science, a k-d tree (short for k-dimensional tree) is a space-partitioning data structure for organizing points in a k-dimensional space. With the help of AI, the researchers could narrow their search for space abnormalities.
Also Read : Rapidly Expanding Black Hole Discovered using ANU's SkyMapper Telescope, Devours Planets
What are the Space Anomalies?
In the past few years, astronomers have investigated astronomical objects by examining their light curves, illustrating how an object's brightness changes over time. They first recognize a beam of light in the sky and then track its progression to determine if the light becomes brighter, dimmer, or fades over time.
Per Phys.org, these researchers evaluated a million genuine light curves from the ZTF's 2018 collection as well as seven simulated live curve models of the sorts of items under review in their study. So far, they tracked 40 factors in all, including the intensity of an object's brightness and its duration.
They specified the qualities of their simulations using a set of characteristics predicted to be detected in genuine celestial bodies, according to co-author Konstantin Malanchev. As for their purpose, they were seeking super-powerful supernovae, Type Ia supernovae, Type II supernovae, and tidal disruption events in the collection of nearly a million items.
"We refer to such classes of objects as anomalies. They are either very rare, with little-known properties, or appear interesting enough to merit further study,' said Malanchev.
Using k-D tree algorithm, they found 15 nearest neighbors, i.e. actual items from the ZTF database, for each simulation-a total of 105 matches, which the researchers then visually checked for irregularities. The manual analysis validated 11 anomalies, seven of which were supernova prospects, and four were galactic nuclei candidates for tidal disruption scenarios.
Maria Pruzhinskaya, a co-author of the paper and research fellow at the Sternberg Astronomical Institute, said, "In addition to the already-discovered rare objects, we were able to detect several new ones previously missed by astronomers. This means that existing search algorithms can be improved to avoid missing such objects."
This study reveals that SNAD's k-D tree and AI machine algorithm strategy is successful and reasonably simple to implement. The suggested technique for identifying certain forms of space events is universal and may be used to find any fascinating astronomical phenomenon, not just unusual varieties of supernovae.
Related Article : Orbital Issues Experienced by Momentus: Space Tugs Error
This article is owned by Tech Times
Written by Thea Felicity
ⓒ 2025 TECHTIMES.com All rights reserved. Do not reproduce without permission.