According to a recent study, artificial intelligence (AI) is capable of accurately identifying a person's race from radiographic scans, a feat that human experts are unable to replicate.
As reported first by Interesting Engineering, the study suggests that the integration of race into image analysis could cause further racial bias and disparities in the medical field.
The first study author and NIBIB Data and Technology Advancement (DATA) National Service Scholar Judy Gichoya, M.D., acknowledged that AI has immense potential in transforming the treatment of several diseases and conditions. However, she also stressed that there is a need to understand how AI algorithms work to ensure that it benefits all patients.
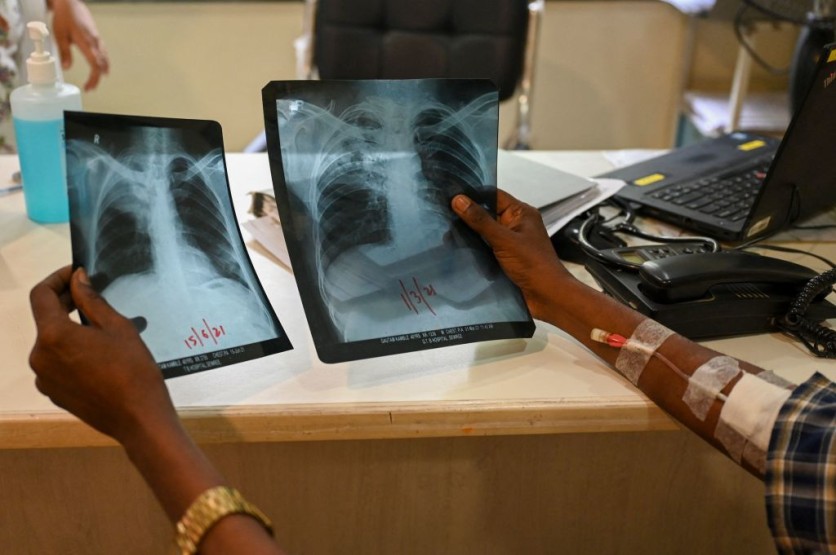
Bias in AI Systems
Bias in AI systems is not a brand-new concept. Studies have revealed that demographic factors, like race, can have an impact on AI's performance.
The use of datasets that are not representative of the patient community, such as those where the majority of patients are white, is one of many potential reasons that could result in bias in AI systems, as noted by the study's press release.
Additionally, the study claims that bias can be introduced by confounders, which are features or phenotypes that are disproportionately prevalent in subgroup populations, such as ethnic variations in breast or bone density.
Another potential element that can induce unintentional biases in AI algorithms is highlighted by the current study via X-ray scans.
Startling Discovery
Gichoya and colleagues first sought to see if they could create AI models that could identify race only from chest X-rays for their investigation. They found that their algorithms could predict race with a high degree of accuracy using three huge datasets that covered a diverse patient population.
This was a startling discovery because human specialists are unable to make such predictions by studying X-rays.
The researchers also discovered that the AI was able to identify the self-reported race even when the resolution was significantly altered, the photos were cropped to only one-ninth of their original size, or the images were scarcely identifiable as X-rays.
The research team subsequently tested the AI's ability to identify self-reported race using a variety of non-chest X-ray datasets, such as chest computer tomography (CT) scans, mammograms, and cervical spine radiographs, and discovered that it was unaffected by the type of scan or anatomic location.
"Hidden Signals"
"Our results suggest that there are 'hidden signals' in medical images that lead the AI to predict race," Gichoya said in a statement.
The researchers also looked at a wide range of variables, including body mass index (BMI), bone density, breast density, and disease distribution, that might have an impact on features in radiography images.
They were unable to pinpoint any particular element that would account for how AI might correctly predict the self-reported race. In other words, while AI may be taught to infer race from medical imaging, it is yet unknown what data the models utilize to do so.
"There has been a line of thought that if developers 'hide' demographic factors-like race, gender, or socioeconomic status-from the AI model, that the resulting algorithm will not be able to discriminate based on such features and will therefore be 'fair.' This work highlights that this simplistic view is not a viable option for assuring equity in AI and machine learning," NIBIB DATA Scholar Rui Sá, Ph.D., said in a statement.
This article is owned by Tech Times
Written by Joaquin Victor Tacla
ⓒ 2025 TECHTIMES.com All rights reserved. Do not reproduce without permission.