Bias in algorithms have always been a key issue in the growing field of artificial intelligence. For instance, researchers found in an experiment that flawed AI in robots has the tendency to make racial and gender stereotypes. Another study argues that AI hiring tools have failed to reduce bias and that they are only equivalent to "automated pseudoscience."
To address this issue, some developers are making sure that their algorithms will not pose harmful biases especially in the fields of banking, healthcare, and many more. These are called "fairness" algorithms.
Now, a group of researchers from the University of Southern California (USC) Viterbi School of Engineering have developed FairFed, a fairness enhancing algorithm that also promises to keep data secured.
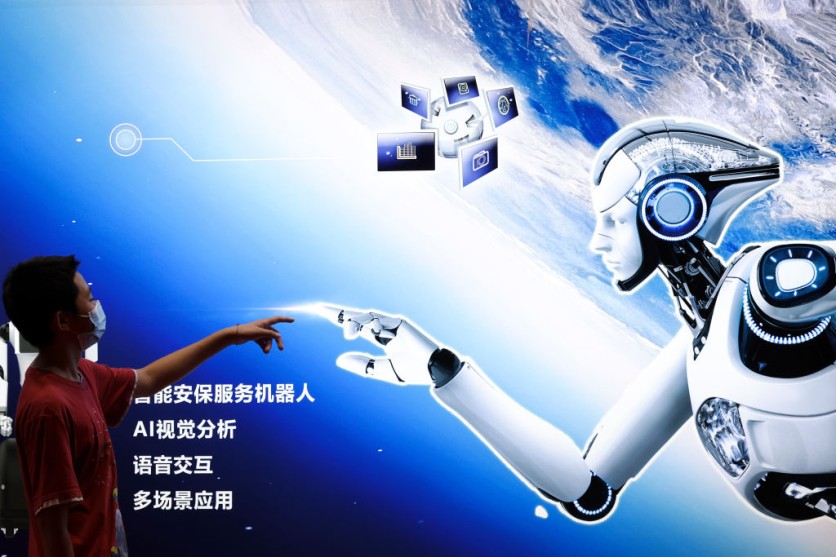
Federated Learning
According to the research team, debiasing information sources can reduce bias in machine learning (ML) algorithms. However, the source data is not always accessible.
This is evident in federated learning where an ML method is utilized to train algorithms in various decentralized datasets without the need of exchanging local data samples.
"Federated learning has been viewed as a promising solution for collaboratively training machine learning models among multiple parties while maintaining their local data privacy," Shen Yan, the study's co-author, said in a statement.
"However, federated learning also poses new challenges in mitigating the potential bias against certain populations (e.g., demographic groups), as this typically requires centralized access to the sensitive information (e.g., race, gender) of each datapoint."
Hence, FairFed was developed to improve fairness in federated learning.
How Does FairFed Work?
Local debiasing is carried out by each entity on FairFed's data set. By using information about the local population, they debias their algorithm.
These entities determine a local fairness metric, which assesses the algorithm's fairness to the local population.
The entities then assess the fairness of the global model on their local datasets and work with the server to change its model aggregation weights and improve the local debiasing performance.
The results of the algorithm have so far delighted the research team. They claim that FairFed offers a practical and successful method for enhancing federated learning systems.
"Heterogeneous data," or data having a wide variety of forms and formats, are present in real-world decision-making situations. Hence, the team used diverse data to analyze FairFed.
Under conditions of high data heterogeneity, they discovered that FairFed performed better than cutting-edge fair federated learning frameworks, ensuring that the results were more equitable for various demographic groups.
Yan claimed that during the aggregation phase, they managed to debias the federated learning system. The researcher notes that this will ensure that the system will not access an individual's data and make fair decisions as well.
The team will further discuss their findings on the upcoming 37th AAAI Conference on Artificial Intelligence, an event that promotes research in the field of AI.
Related Article : Fraud Security Startup Hawk AI Raises $17 Million to Expand its Products for Banks, Payment Firms, and Fintechs
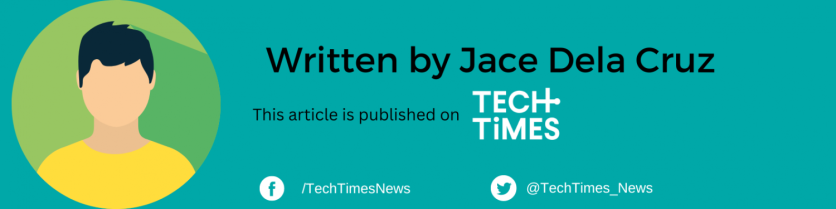
ⓒ 2025 TECHTIMES.com All rights reserved. Do not reproduce without permission.