In a pioneering effort, researchers have introduced a novel technique to enhance the resilience of artificial neural networks (ANNs) by incorporating random noise into their inner layers.
According to TechXplore, ANNs, similar to the structure of the human brain, are fundamental to various AI systems. However, like the human brain, ANNs can occasionally misinterpret inputs, potentially leading to critical errors in decision-making processes.
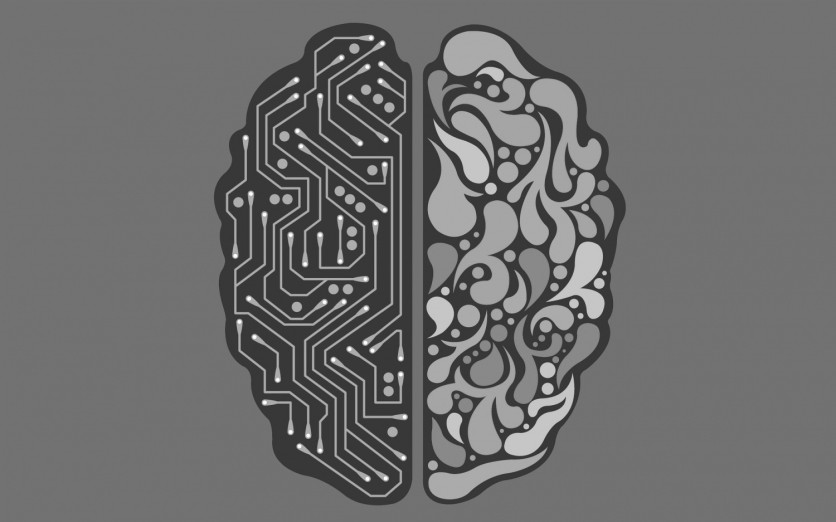
Introducing Random Noise in Deeper Layers of Network
This novel approach, crafted by recent graduate Jumpei Ukita and Professor Kenichi Ohki from the Department of Physiology at the University of Tokyo Graduate School of Medicine, introduces random noise not only at the input layer but also in deeper layers of the network.
This is a practice typically avoided due to concerns about its impact on normal functioning. However, the duo found that this noise actually heightened the network's adaptability without compromising its regular performance.
The result was a marked reduction in susceptibility to simulated adversarial attacks, demonstrating the efficacy of their method. Ukita emphasizes that the ongoing arms race between attackers and defenders in the realm of AI systems necessitates continuous innovation to safeguard the systems that are integral to our daily lives.
This research signifies a significant leap forward in fortifying the reliability and security of artificial neural networks. Artificial intelligence has permeated various aspects of modern life, from voice assistants on smartphones to search engines powered by intricate AI algorithms.
These systems are predominantly constructed using ANNs, which are modeled after the structure of the human brain. While they can process immense amounts of data and make decisions, they can sometimes be confounded, either accidentally or by the deliberate actions of external entities.
Arms Race Between Attackers and Defenders
Unlike human perception, an ANN might interpret seemingly ordinary visual inputs in entirely different ways. For instance, a medical diagnostic system could erroneously identify a healthy condition as a medical issue.
Such discrepancies pose significant challenges, especially in autonomous vehicles and medical diagnostics applications. While defenses against such attacks exist, they have their limitations.
Hence, this is the reason why the new approach was devised. They drew inspiration from their background in studying the human brain.
"These attacks work by supplying an input intentionally far from, rather than near to, the input that an ANN can correctly classify. But the trick is to present subtly misleading artifacts to the deeper layers instead," Ukita said in a press statement.
"Once we demonstrated the danger from such an attack, we injected random noise into the deeper hidden layers of the network to boost their adaptability and therefore defensive capability. We are happy to report it works," Ukita added.
The researchers observed a reduction in susceptibility to simulated adversarial attacks, showcasing the efficacy of their method. However, they acknowledge the need for further development to make it more robust against a wider range of attacks.
Ukita emphasizes the ongoing arms race between attackers and defenders of AI systems. He underscores the necessity for continuous innovation to safeguard the systems integral to our daily lives.
This research marks a significant stride in fortifying the reliability and security of artificial neural networks. The findings of the team were published in the journal Neural Networks.
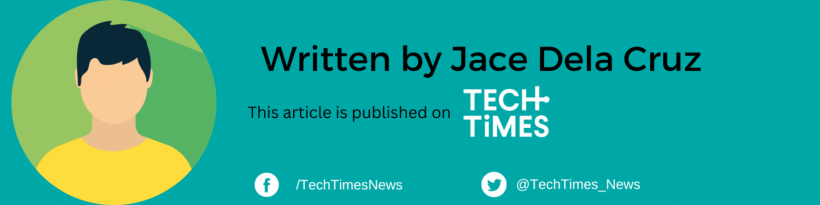
ⓒ 2025 TECHTIMES.com All rights reserved. Do not reproduce without permission.