MIT researchers are pioneering a novel approach to train machine learning models by utilizing synthetic imagery, surpassing the efficacy of traditional methods relying on real images.
The key to this breakthrough lies in implementing StableRep, a system that leverages text-to-image models, particularly Stable Diffusion, to generate synthetic images through a technique known as "multi-positive contrastive learning."

All About the StableRep
Lijie Fan, a PhD student in electrical engineering at MIT and lead researcher on the project, explained the methodology, saying that instead of merely feeding data to the model, StableRep employs a strategy that teaches the model to comprehend high-level concepts through context and variance.
"When multiple images, all generated from the same text, all treated as depictions of the same underlying thing, the model dives deeper into the concepts behind the images, say the object, not just their pixels," Fan said in a press statement.
The system considers multiple images generated from the same text prompt as positive pairs, guiding the model to delve deeper into conceptual understanding rather than focusing solely on pixel-level details.
This innovative approach has demonstrated superior performance to top-tier models trained on real images, such as SimCLR and CLIP. StableRep addresses challenges related to data acquisition in machine learning and represent a stride toward more efficient AI training techniques.
By producing high-quality synthetic images on demand, the system has the potential to reduce the cumbersome expenses and resources associated with traditional data collection methods.
The MIT team noted that the evolution of data collection in machine learning has seen significant challenges. From manual capture of photographs in the 1990s to internet scouring for data in the 2000s, the process has been labor-intensive and prone to discrepancies.
According to the researchers, raw, uncurated data often contains biases and does not faithfully represent real-world scenarios.
Read Also : MIT Unveils F3RM: AI-Powered Robots Can Now Grasp Unfamiliar Objects Using Natural Language
'Guidance Scale'
StableRep's success is attributed, in part, to the fine-tuning of the "guidance scale" in the generative model, striking a balance between diversity and fidelity of synthetic images. The system's reported effectiveness challenges the notion that vast real-image collections are indispensable for training machine learning models.
An enhanced variant, StableRep+, introduced language supervision into the training process, further improving accuracy and efficiency. However, the researchers acknowledge certain limitations, including the slow pace of image generation, potential biases in text prompts, and challenges in image attribution.
While StableRep represents a significant advancement by reducing reliance on extensive real-image collections, concerns about hidden biases in uncurated data and the importance of meticulous text selection persist.
The researchers emphasize the need for ongoing improvements in data quality and synthesis, recognizing the system's contribution as a step forward in cost-effective training alternatives for visual learning.
"Our work signifies a step forward in visual learning, towards the goal of offering cost-effective training alternatives while highlighting the need for ongoing improvements in data quality and synthesis," Fan noted.
The findings of the team were published in arXiv.
Related Article : MIT Takes Strides Towards Greener AI: Addressing the Environmental Impact of Energy-Intensive Models
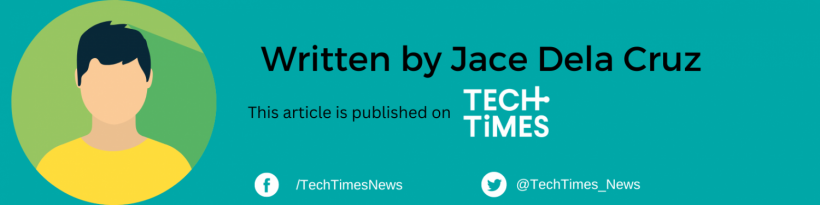
ⓒ 2025 TECHTIMES.com All rights reserved. Do not reproduce without permission.