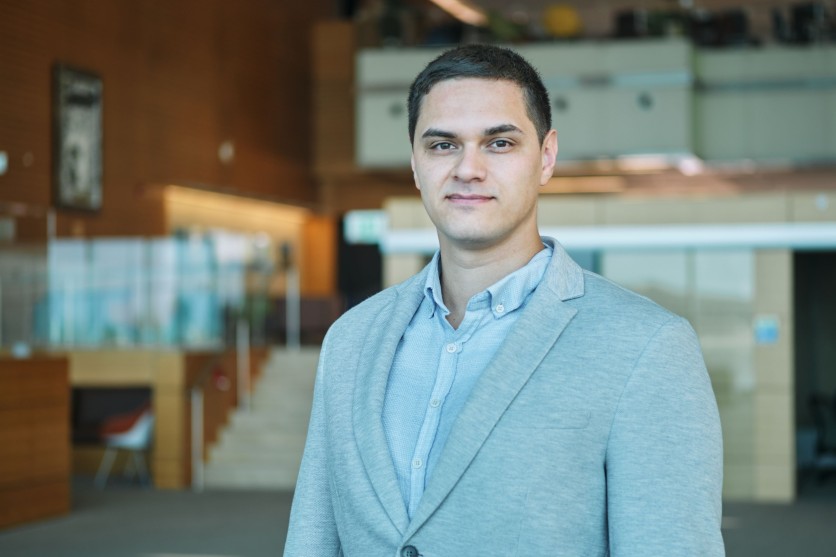
I met Dmitrii Khizbullin at ICML 2024 in Vienna. While we began talking about large language models, the conversation quickly turned to agents—those emergent, sometimes chaotic, and increasingly sophisticated AI systems that act with a semblance of autonomy. It's a field in which Khizbullin has quietly become one of the leading and most intriguing minds.
A trained physicist turned AI engineer, Khizbullin has built a career around designing and deploying intelligent systems in both industrial and academic settings. However, his recent contributions focus sharply on AI agents and how to make them more robust, collaborative, and evaluative. "We're moving from prompting to orchestrating," he told me, describing the shift in mindset required to design agentic systems.
At the core of that shift is "CAMEL: Communicative Agents for 'Mind' Exploration of Large Language Model Society," a 2023 NeurIPS paper that Khizbullin co-authored with a team led by Bernard Ghanem, a prominent professor at KAUST. The paper, which has already been cited nearly 700 times, was one of the first to simulate multi-agent societies built entirely from LLMs and explore how communication and personality shape emergent behavior. "CAMEL made it fun," he said. "We showed that language models can simulate complex social interactions—and sometimes surprise you with how reminiscent they are of their human prototypes."
Khizbullin didn't stop there, though. While at ICML 2024, he co-authored "GPTSwarm: Language Agents as Optimizable Graphs." This work, done in collaboration with AI pioneer Jürgen Schmidhuber, framed agents not just as scripts but as nodes in a graph that can be optimized. "It opened the door to agent-level learning," he explained. "You can not only tune the models but how they interact, too."
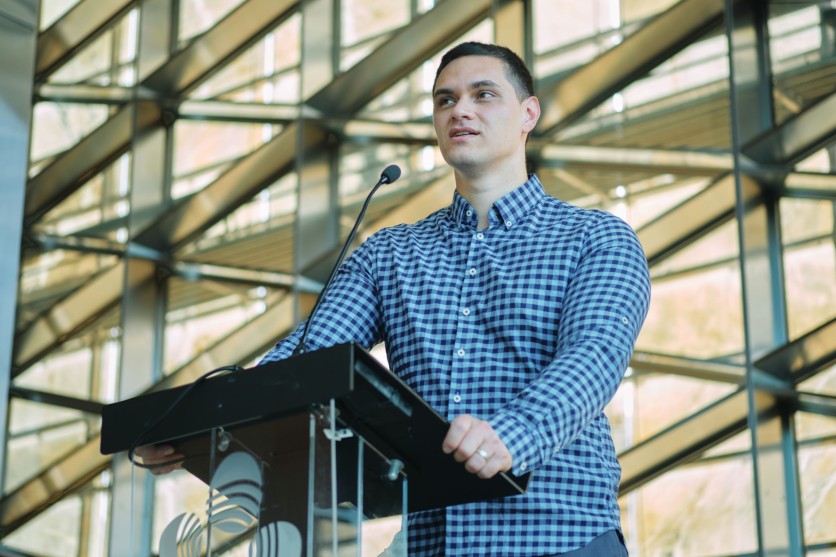
That trajectory has continued into 2025. A new paper, "Agent-as-a-Judge: Evaluate Agents with Agents," is making its way to ICML 2025. It proposes that LLM-based agents can be used to evaluate other agents, creating a kind of recursive loop that could transform how we benchmark the performance of AI agents. "Evaluation is hard," Khizbullin said. "But if we want to scale agent systems, we need tools that understand nuance."
Another recent preprint, "How to Correctly Do Semantic Backpropagation on Language-Based Agentic Systems," pushes further into training and optimization, suggesting mechanisms to make language-based agents more focused on goals and outcomes. "It's about control and feedback," he noted. "Agents that can learn not just from their own mistakes, but from a wider context of the problem as well."
Even in his latest work, Khizbullin stays within the agentic paradigm. His 2025 paper, "Beyond Outlining: Heterogeneous Recursive Planning for Adaptive Long-Form Writing with Language Models," introduces an AI agent capable of sophisticated recursive planning. The system dynamically adjusts its goals and structure as it writes, resembling a cognitive process rather than a rigid language model response. Remarkably, the agent can generate both vivid, engaging fiction and detailed, factually grounded reports, on par with tools like OpenAI's Deep Research or Manus. I tried it myself and found the storytelling both coherent and surprisingly compelling. "It's a writing agent," he explained. "Not just generating content, but planning, revising, and adapting just like a human author."
Khizbullin collaborates closely with Jürgen Schmidhuber, considered by many as one of the godfathers of AI, and Bernard Ghanem, a key figure in applied AI research. It's a rare combination of theoretical depth and production focus brought together in one AI engineer.
"There's a lot of hype in this space," he said as our chat wound down. "But AI agents are here to stay. The question is how we design them to be useful, stable, and trustworthy."
Khizbullin is part of a unique group of engineers who move easily between publishing papers and shipping code. As AI systems grow more agentic, his work may not only help define how they think but also how we think about them.
ⓒ 2025 TECHTIMES.com All rights reserved. Do not reproduce without permission.