The two most popular techniques for quick COVID-19 testing are serology and molecular tests. Serology tests look for the presence of antibodies brought on by the SARS-CoV-2 virus, and molecular testing detects the existence of viral SARS-CoV-2 RNA.
According to Florida Atlantic University's press release, there is currently no research on the correlation between molecular and serology tests and which particular COVID-19 symptoms are indicative of a positive test result.
But with the help of machine learning, a study from Florida Atlantic University's College of Engineering and Computer Science offers crucial new evidence for identifying how molecular tests and serology tests are correlated as well as which features are most effective at differentiating between COVID-19 positive and negative test results.
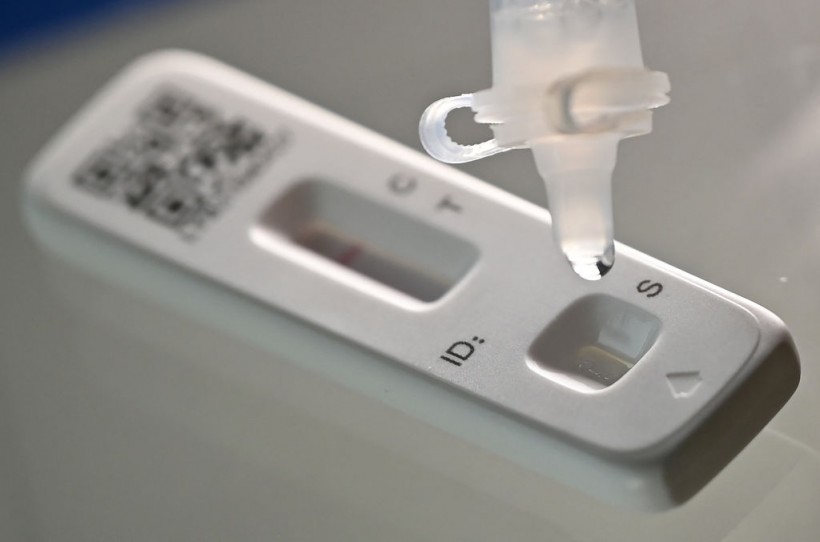
(Photo : JUSTIN TALLIS/AFP via Getty Images)
An illustration picture taken on February 20, 2022 shows a model using a SARS-CoV-2 Antigen Rapid Test contained in a Covid-19 Lateral Flow (LFT) self-test kit, in London.
AI Predictive Model
To forecast the outcomes of the COVID-19 test, the researchers trained five classification algorithms. They used easily accessible symptom features, combined with demographic information such as age, gender, fever, and the number of days from the onset of the symptoms, to construct an accurate predictive model.
The study shows that machine learning models can predict COVID-19 infections when they are trained using basic symptom and demographic variables.
The findings, which were published in the journal Smart Health, pinpoint the essential symptoms linked to COVID-19 infection and offer a method for quick screening and affordable infection diagnosis.
Additionally, research indicates that when compared to post-symptom onset days of serology tests between five to 38 days, molecular tests have substantially narrower post-symptom onset days which are three and eight days.
They discovered that the molecular test had the lowest positive rate because it detects an ongoing infection.
The team also noted that there are substantial differences amongst COVID-19 tests since donors' immune responses and viral loads, which are the focus of many test methodologies, are always changing.
Hence, it may be possible to see various positive/negative results from the two different types of tests, even for the same donor.
Read Also: COVID-19 Mutations Found in NYC Rats; Can These Rodents Infect Humans? Officials Share Concerns
The Study's Testbed
The research team used test results from 2,467 donors who were each subjected to one or more COVID-19 tests as the testbed for the study.
They created a set of characteristics for predictive modeling utilizing the five different types of machine-learning models by combining symptoms and demographic data.
They looked into the link between serology and molecular tests by comparing test types and outcomes. They used the serology or molecular test findings to categorize the 2,467 donors as positive or negative for test outcome prediction, and they developed symptom features to represent each donor for machine learning.
These predictive models achieved AUC scores of more than 81 percent and classification accuracy of more than 76 percent by combining produced bin features with the five machine-learning techniques.
"Our researchers have designed a new way to narrow down noisy symptom features for clinical interpretation and predictive modeling," Stella Batalama, Ph.D., Dean, FAU College of Engineering and Computer Science said in a statement.
"Such AI based predictive modeling approaches are becoming increasingly powerful to combat infectious diseases and many other aspects of health issues."
Related Article: COVID-19 Rapid Tests Still Efficient Against New Sub-Variants, Experts Claim But There's a Catch