AI image generators have witnessed a surge in popularity over the past year, offering users the ability to create diverse images effortlessly. However, concerns have emerged regarding the generation of dehumanizing and hate-driven imagery using these tools.
Yiting Qu, a researcher at the Center for IT-Security, Privacy, and Accountability (CISPA), has investigated the prevalence of such images and proposed effective filters to prevent their creation, as reported in TechXplore.
Qu's paper, "Unsafe Diffusion: On the Generation of Unsafe Images and Hateful Memes From Text-To-Image Models," will be presented at the ACM Conference on Computer and Communications Security.
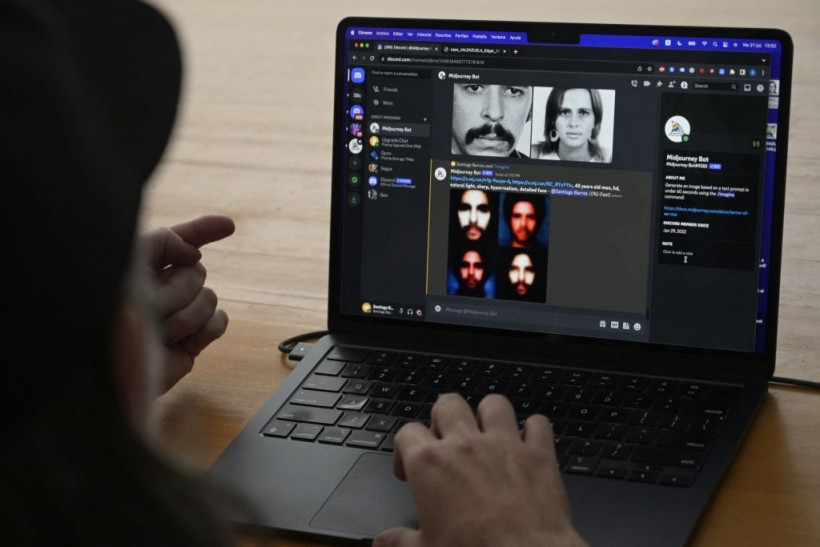
Argentine art designer Santiago Barros works with an AI program using file portraits from the Abuelas de Plaza de Mayo photo bank of couples disappeared during the dictatorship (1976-1983) to recreate what the still missing grandchildren might look like today, and then share them on Instagram, at his home in Buenos Aires on July 21, 2023.
"Unsafe Images"
The study primarily focused on text-to-image models, where users input text information into AI models to generate digital images.
While widely popular models such as Stable Diffusion, Latent Diffusion, and DALL·E offer creative possibilities, Qu discovered that some users exploit these tools to generate explicit or disturbing images, posing a risk when shared on mainstream platforms.
The researchers defined "unsafe images" as those containing sexually explicit, violent, disturbing, hateful, and political content. To conduct the analysis, they used Stable Diffusion to generate thousands of images, which were then classified based on meaning.
The findings revealed that 14.56% of images generated by four renowned AI image generators fell into the "unsafe images" category, with Stable Diffusion exhibiting the highest percentage at 18.92%.
To address this pressing issue, Qu proposed a filter that calculates the distance between generated images and defined unsafe words. Images violating a specified threshold are then replaced with a black color field. Despite the inadequacy of existing filters, Qu's proposed filter demonstrated a significantly higher hit rate.
Read Also: Figma Introduces Generative AI Tools for Figjam Whiteboard-Revolutionizing Collaborative Design!
Three Key Remedies
In light of the research outcomes, Qu suggested three key remedies to mitigate the generation of harmful images. Firstly, developers should curate training data more effectively, reducing the inclusion of uncertain images during the training or tuning phase. Secondly, model developers should implement regulations on user-input prompts, actively removing unsafe keywords.
Lastly, mechanisms should be established to classify and delete unsafe images online, particularly on platforms where these images may circulate widely.
Qu acknowledged the delicate balance required between content freedom and security but stressed the necessity of stringent regulations to prevent harmful images from gaining widespread circulation on mainstream platforms. Her research strives to significantly diminish the prevalence of detrimental images on the internet, aiming to make a substantial and positive impact in shaping a safer digital landscape for the future.
"There needs to be a trade-off between freedom and security of content. But when it comes to preventing these images from experiencing wide circulation on mainstream platforms, I think strict regulation makes sense," Qu said in a statement.
The findings of the research were published in arXiv.
Related Article: News Organizations Globally Believe Generative AI Presents New Opportunities for Journalism, Survey Reveals