Artificial intelligence (AI) predictive models, a cornerstone of modern healthcare, might face unforeseen challenges, suggests a study by researchers at the Icahn School of Medicine and the University of Michigan.
According to MedicalXpress, the research delves into the implications of implementing predictive models on their subsequent performance. It reveals that their utilization in adjusting healthcare delivery could alter underlying assumptions, potentially to the detriment of patient care.
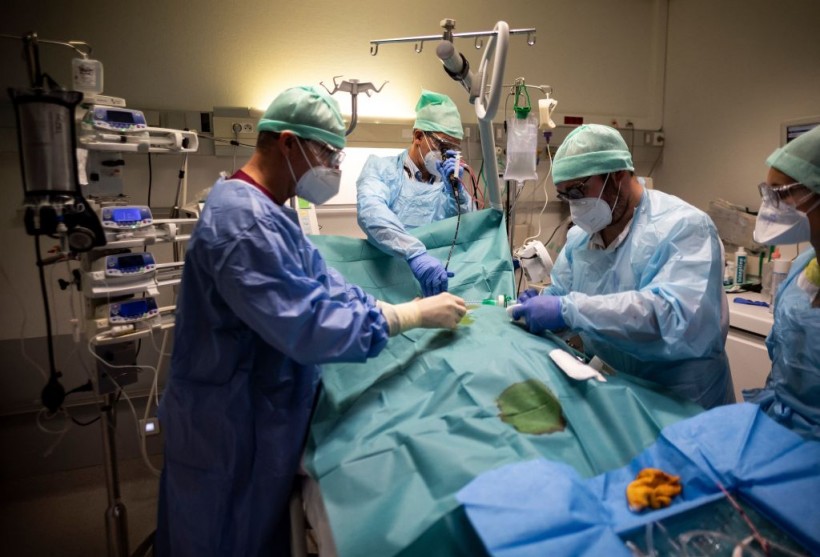
Medical personnel attend a covid-19 patient at the intensive care unit of the clinic of Occitanie in Muret, near Toulouse, southern France on November 17, 2020. Government officials have indicated they hope to ease the lockdown from next month if the health crisis eases, in particular for hospitals dealing with a surge in intensive care patients.
130,000 Critical Care Admissions
The study simulated critical care scenarios at major healthcare institutions, analyzing 130,000 critical care admissions. The researchers explored three key scenarios.
First, the practice of retraining models to combat performance degradation over time was assessed. While retraining initially improved performance by adapting to changing conditions, the study indicated that it could paradoxically lead to degradation by disrupting learned relationships between presentation and outcome.
Second, following a model's predictions to prevent adverse outcomes, such as sepsis, was considered. However, the study noted that this approach could inadvertently lead to death after sepsis.
Additionally, it highlighted that using machine-learning-influenced care data might be unsuitable for training future models due to the unpredictability of outcomes.
Lastly, the study examined the impact of simultaneous predictions from two models. It was found that using one set of predictions rendered the other obsolete, underscoring the need for fresh data, which can be resource-intensive.
Read Also: MIT Takes Strides Towards Greener AI: Addressing the Environmental Impact of Energy-Intensive Models
Complexities of AI Predictive Models
"Our findings reinforce the complexities and challenges of maintaining predictive model performance in active clinical use," said co-senior author Karandeep Singh, MD, Associate Professor of Learning Health Sciences, Internal Medicine, Urology, and Information at the University of Michigan.
"Model performance can fall dramatically if patient populations change in their makeup. However, agreed-upon corrective measures may fall apart completely if we do not pay attention to what the models are doing-or more properly, what they are learning from," Singh added.
The research emphasizes the intricate challenges of maintaining predictive model performance in active clinical use. It points out that shifts in patient populations could lead to a dramatic drop in model performance.
The study urges healthcare systems to establish systems to track individuals affected by machine learning predictions and calls for governmental agencies to issue relevant guidelines. The authors caution against such models' indiscriminate use and updates, as they may lead to false alarms, unnecessary testing, and increased costs.
The findings underscore the need for a thoughtful, nuanced approach to deploying predictive models in healthcare. The study asserts that while predictive models are valuable tools, they require regular maintenance, contextualization, and understanding to ensure their effectiveness.
The findings of the team were published in the ACP Journals.
Related Article: New Study Delves Into World of Artificial Neural Networks, Unraveling How AI Brains Learn