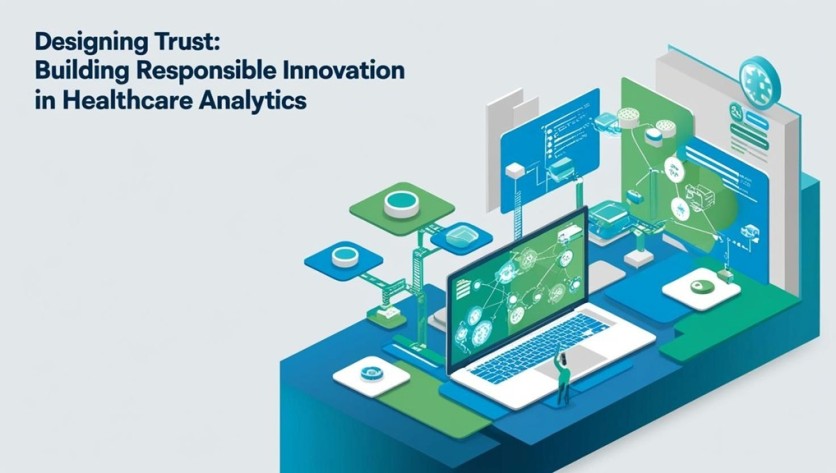
As healthcare systems increasingly rely on data-driven insights, ensuring ethical and regulatory compliance has become crucial. This article explores how privacy, transparency, and fairness are being embedded into healthcare analytics, drawing on recent work by researcher Venkat Mounish Gundla in the evolving compliance landscape. His insights offer a timely guide for responsible innovation in healthcare data science.
The New Frontier of Predictive Healthcare
The exponential growth of predictive analytics in healthcare marks a pivotal shift in patient care. Projected to grow from $12.5 billion in 2023 to over $72 billion by 2032, the field promises smarter diagnostics, personalized treatments, and data-driven efficiency. Yet, this promise is bound by a paradox: the same data needed for breakthroughs is protected under some of the world's strictest privacy laws. As systems lean into AI and analytics, the challenge is clear: innovation must operate within a lattice of regulatory frameworks that evolve as quickly as the technology itself.
Charting the Regulatory Maze
Two influential privacy regulations, HIPAA and GDPR, form the backbone of healthcare data compliance. In the U.S., HIPAA's 2024 cybersecurity rules demand AI-specific risk assessments and broaden breach notification requirements. Annual penalties now reach $1.8 million, reinforcing the cost of non-compliance. Across the Atlantic, GDPR frames healthcare as high-risk. Improper data handling and weak security have led to over €173 million in fines. As laws layer atop international frameworks, harmonization becomes a hurdle, especially for cross-border data flows.
Privacy by Architecture: The Building Blocks
At the core of compliant systems lie key technical components:
- Data Classification: Locating sensitive information supports proportional security.
- De-identification: Removing identifiers lowers breach impact.
- Secure Storage and Access Controls: Encryption and audit logs detect incidents faster.
- Consent Management: Consent-tracking systems uphold user autonomy.
A "compliance-by-design" mindset embedding these elements from the outset proves more efficient than retroactive fixes.
Innovation Within Guardrails
Compliance is more than following rules; it means designing systems that respect ethics. Techniques like synthetic data, differential privacy, and federated learning maintain utility while protecting identities. New AI regulations, like the EU AI Act, require fairness assessments and transparency. Organizations that build fairness into their workflows cut bias-related issues by over 50%.
Overcoming Old Tech and New Borders
Legacy systems threaten security. Many still run outdated platforms lacking support for modern safeguards. Gateway encapsulation helps, but long-term solutions require risk-guided upgrades. Cross-border operations juggle multiple privacy frameworks. Unified architectures have reduced documentation and audit prep time by over 70%. These approaches ensure consistency while reducing compliance overhead across jurisdictions.
Ethical Data Use Beyond the First Purpose
Secondary data use demands ethical governance. Tools like purpose assessments and tiered consent enable broader yet responsible reuse. Structured governance leads to fewer complaints and better research outcomes. Explainability also drives adoption. Algorithms with transparent outputs enjoy greater clinician trust and higher approval rates. Patients are more likely to accept AI-assisted decisions when they understand how they're made.
Empowering Patients Through Transparent Systems
Data-driven healthcare and transparency are emerging as a cornerstone of trust. Patients are increasingly aware of how their data is used and demand clarity around automated decision-making. Systems that offer tiered explanations of AI outcomes tailored for clinicians, administrators, and patients demonstrate higher acceptance and satisfaction rates. Such systems also see improved clinical integration, as explainable models are more readily endorsed by practitioners. Furthermore, clear documentation of data provenance and model limitations is now a regulatory expectation, reinforcing the need for communication strategies that bridge the gap between complex algorithms and real-world impact.
Creating Ethical Foundations for Future Innovation
As analytics and AI capabilities continue to evolve, ethics must remain at the forefront. This includes ensuring equitable outcomes across demographic groups, establishing fair secondary use policies, and embedding oversight throughout the system lifecycle. Ethical governance structures such as AI ethics committees, formal review processes, and inclusive stakeholder engagement enable organizations to innovate responsibly. Rather than stifle progress, these frameworks guide development toward socially beneficial outcomes, balancing technological potential with human dignity and rights.
In conclusion, as healthcare analytics evolves, Venkat Mounish Gundla's insights provide a roadmap for integrating compliance into innovation. The message is clear: protecting privacy is not just a legal obligation, it is a foundational principle for advancing healthcare in a trustworthy, effective, and ethical manner.
ⓒ 2025 TECHTIMES.com All rights reserved. Do not reproduce without permission.