Machine-learning models, often used to make decisions about rule violations, are failing to replicate human judgment, according to a study conducted by researchers from MIT and other institutions.
The study found that when not trained with the right data, these models tend to make different and often harsher judgments than humans.
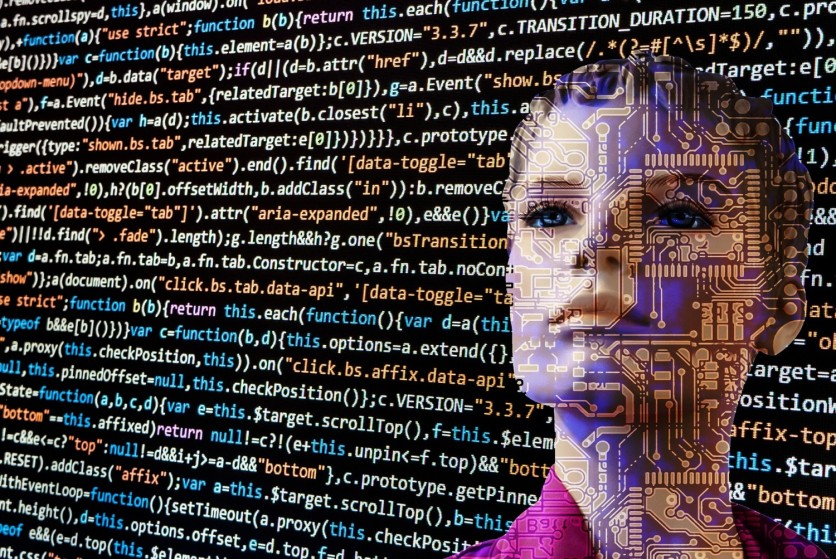
The Key Issue
The key issue lies in the data used to train the machine-learning models. Typically, the data is labeled descriptively, where humans are asked to identify factual features.
For example, in the case of judging whether a meal violates a school policy that prohibits fried food, humans are asked to determine the presence of fried food in a photo.
However, when these descriptive models judge rule violations, they tend to over-predict such violations.
The implications of this drop in accuracy are significant. For example, suppose a descriptive model is utilized to evaluate the probability of an individual committing another offense. In that case, the study indicates that it might impose more stringent judgments compared to those made by humans. Consequently, this could lead to elevated bail amounts or lengthier sentences for criminals.
According to Marzyeh Ghassemi, an assistant professor and head of the Healthy ML Group in MIT's Computer Science and Artificial Intelligence Laboratory (CSAIL), these models fail to replicate biased human judgments because the training data itself is flawed.
If humans were aware that their labels would be used for judgments, they would label images and text differently. This has significant implications for machine learning systems integrated into human processes.
Labeling Discrepancy
The research team conducted a user study to investigate the labeling discrepancy between descriptive and normative labels. They gathered four datasets to mimic different policies and asked participants to provide descriptive or normative labels.
The results showed that humans were likelier to label an object as a violation in the descriptive setting. The disparity ranged from 8 percent for dress code violations to 20 percent for dog images.
To further explore the impact of using descriptive data, the researchers trained two models-one using descriptive data and the other using normative data to judge rule violations.
The examination indicated that the model trained using descriptive data performed less effectively than the model trained using normative data.
The descriptive model demonstrated a greater tendency to misclassify inputs by inaccurately predicting rule violations. Moreover, its accuracy significantly decreased when categorizing objects that generated disagreements among human labelers.
Dataset Transparency
To tackle this problem, dataset transparency must be enhanced, enabling researchers to understand the data collection process and utilize them correctly.
Another solution is fine-tuning descriptively trained models with a small portion of normative data, a technique known as transfer learning.
The researchers intend to investigate this approach in future studies. Additionally, they have plans to conduct a similar study involving expert labelers to examine the presence of label disparities.
Ghassemi emphasizes the need for transparency in acknowledging the limitations of machine-learning models.
She stated, "The way to fix this is to transparently acknowledge that if we want to reproduce human judgment, we must only use data that were collected in that setting. Otherwise, we are going to end up with systems that are going to have extremely harsh moderations, much harsher than what humans would do. Humans would see nuance or make another distinction, whereas these models don't."
The study was published in the journal Science Advances.
Related Article : Body Clock Problems? New Machine Learning May Improve Sleep
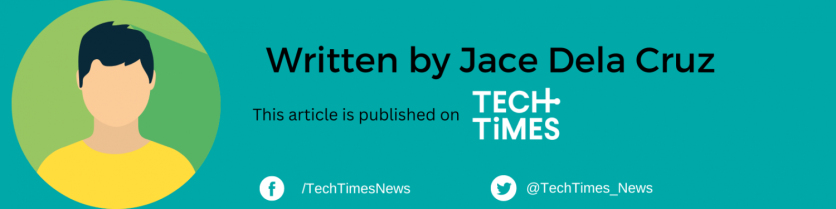
ⓒ 2025 TECHTIMES.com All rights reserved. Do not reproduce without permission.