AI is increasingly being integrated into the healthcare system, promising improved diagnostic accuracy and improved patient care. However, a groundbreaking study has revealed a troubling aspect of AI in healthcare: its potential to mislead clinicians when biased (via Medical Xpress).
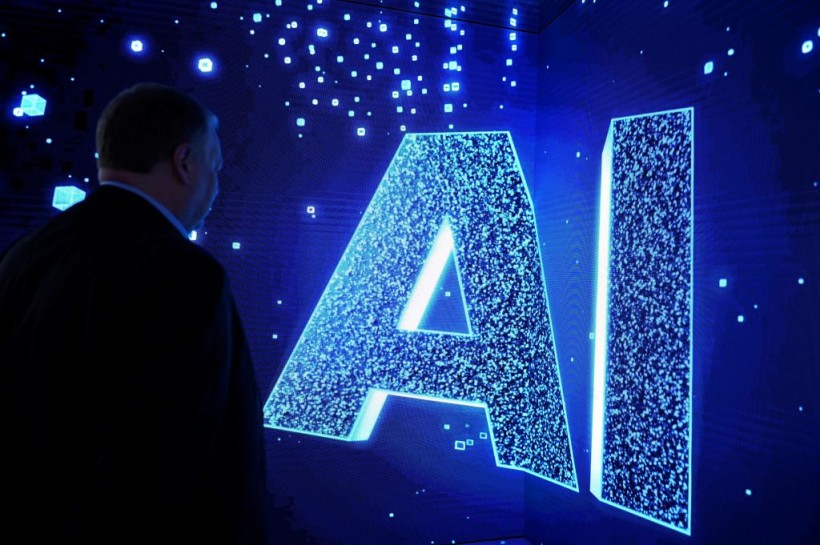
A visitor watches an AI (Artificial Intelligence) sign on an animated screen at the Mobile World Congress (MWC), the telecom industry's biggest annual gathering, in Barcelona.
Impact of AI on Healthcare Professionals
The study, conducted by a multidisciplinary team led by researchers from the University of Michigan, delved into the intersection of AI and clinical decision-making.
Their primary concern? Examining the impact of AI models on diagnostic accuracy among healthcare professionals. Surprisingly, the results were not straightforward; AI models demonstrated a dual nature-a beneficial and a curse.
On the one hand, standard AI models showcased a modest enhancement in clinicians' diagnostic accuracy. When clinicians were provided with AI explanations, their accuracy saw a 4.4% increase compared to baseline scenarios. This revelation hinted at AI's potential to augment clinicians' decision-making processes.
Alarming Findings
However, when investigating biased AI models, the study took a darker turn. These models, which were riddled with systematic errors, posed a significant risk.
They resulted in an alarming 11.3% decrease in clinician diagnostic accuracy, demonstrating the negative effects of biased AI in healthcare.
Jenna Wiens, Ph.D., an associate professor of computer science and engineering and one of the study's co-senior authors, expressed her dissatisfaction with the discovery.
She explained that biased AI models could amplify existing biases in the healthcare system, potentially exacerbating disparities in patient care.
The researchers created scenarios involving patients suffering from acute respiratory failure, a condition notorious for its diagnostic complexities.
Clinicians, including hospitalist physicians, nurse practitioners, and physician assistants, were tasked with diagnosing these cases with and without the assistance of artificial intelligence.
Read Also: Generative AI, Digital Identity Boarding, Forecasted as Aviation's Future
A Closer Look
The pivotal aspect of the study was the role of AI explanations. While they accompanied AI predictions, these explanations failed to rectify the negative impact of biased AI models.
Sarah Jabbour, a Ph.D. candidate in computer science and engineering and the study's first author, emphasized the need for better tools to communicate AI decisions effectively to clinicians.
"The problem is that the clinician has to understand what the explanation is communicating and the explanation itself," Jabbour stated.
Moreover, the study highlighted the importance of regulatory oversight in AI-powered healthcare tools. The US Food and Drug Administration (FDA) has issued guidance to ensure transparency and explainability in AI models used in healthcare. The goal is to empower clinicians to review and comprehend the logic behind AI-driven decisions, mitigating potential risks.
The study's implications reverberate across the healthcare landscape. It raises urgent questions about the safe integration of AI into clinical practice, necessitating further research and development of foolproof AI models devoid of biases.
The need for robust educational initiatives in AI and bias for healthcare professionals has also been underscored.
Stay posted here at Tech Times.
Related Article: AI-Built Cancer Antibodies on the Horizon, Potentially Replacing Chemotherapy with AstraZeneca, Absci Collaboration