A recent study highlights the potential of an AI model in identifying emotional cues like fear and worry in the voices of individuals reaching out to crisis lines, raising prospects for more effective suicide prevention efforts.
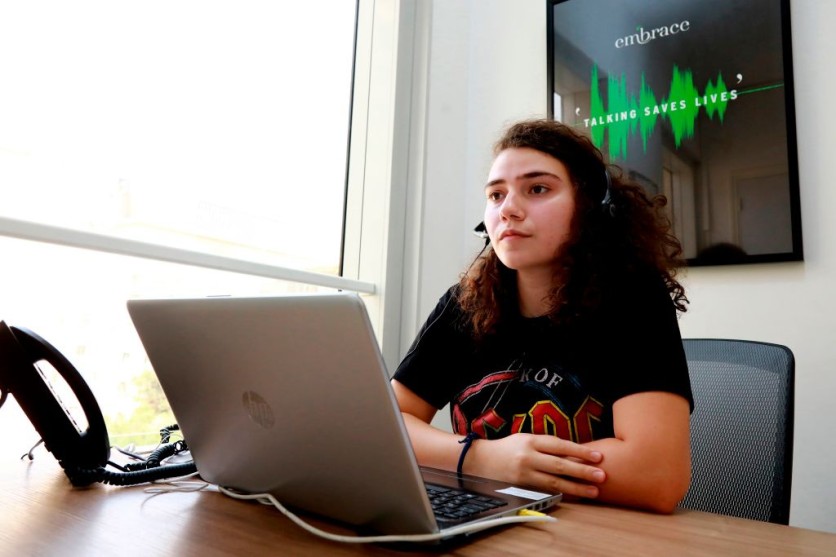
Speech Emotion Recognition (SER) Model
Alaa Nfissi, a PhD student at Concordia University, has developed a novel speech-emotion recognition (SER) model utilizing artificial intelligence tools.
This model analyzes voice waveform modulations to decode emotional states in callers. Nfissi envisions this AI model can significantly enhance responder performance in suicide monitoring scenarios.
Unlike traditional methods reliant on manual annotation by trained psychologists, Nfissi's deep learning model automates the extraction of speech features relevant to emotion recognition, ultimately streamlining the process.
The model's development involved integrating actual calls to suicide hotlines with recordings from actors simulating specific emotions. Trained researchers or the actors themselves annotated these recordings based on the emotions conveyed, enriching the dataset with emotional nuances.
The researcher's deep learning architecture utilizes neural networks, and gated recurrent units process data sequences to extract time-dependent features, enabling the detection of emotional states over a temporal continuum.
One notable improvement in Nfissi's model is its adaptability to varying time segment lengths, eliminating the need for uniform segment durations required by previous models.
Read Also : AI Test Anticipates Knee Osteoarthritis Years in Advance, Offering Hope for Early Intervention
Recognizing Emotions
The model's effectiveness was validated through its ability to accurately recognize emotions within the dataset. It demonstrated proficiency in identifying fearful/concerned/worried emotions 82% of the time, followed by neutral (78%), sad (77%), and angry (72%) emotions.
Notably, the model excelled in identifying professionally recorded segments, achieving success rates ranging from 78% for sad to 100% for angry emotions.
For Nfissi, this research holds personal significance, as it required a deep dive into the intricacies of suicide hotline intervention.
He emphasizes the varying levels of training among counselors and the challenges they face in grasping callers' emotional states quickly.
Nfissi envisions integrating his model into a real-time dashboard for counselors, aiding them in selecting appropriate intervention strategies based on callers' emotional cues, with the ultimate goal of averting potential suicides.
This study underscores the potential of AI-driven speech analysis in augmenting suicide prevention efforts, offering insights into callers' emotional states, and facilitating more targeted intervention strategies.
"Many of these people are suffering, and sometimes just a simple intervention from a counseloor can help a lot. However, not all counsellors are trained the same way, and some may need more time to process and understand the emotions of the caller," Nfissi said in a press release statement.
"This will hopefully ensure that the intervention will help the and ultimately prevent a suicide."
The study, titled "Unlocking the Emotional States of High-Risk Suicide Callers through Speech Analysis," was published in IEEE Xplore.
Related Article : Cambridge's Heartfelt Technologies Introduces AI Telemonitor for Heart Failure Detection Through Foot Volume
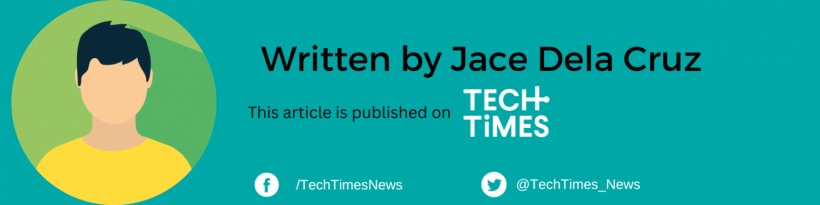
ⓒ 2025 TECHTIMES.com All rights reserved. Do not reproduce without permission.