Machine learning has long drawn inspiration from human learning processes, including the valuable lessons learned from mistakes. However, the application of machine learning in safety-critical autonomous systems, such as self-driving cars and power systems, poses unique risks to human safety.
As the field of machine learning continues to advance, there is a growing interest in addressing these safety concerns in highly complex environments. In a recent study, a groundbreaking research paper challenges the prevailing notion that an unlimited number of trials is necessary to learn safe actions in unfamiliar environments.
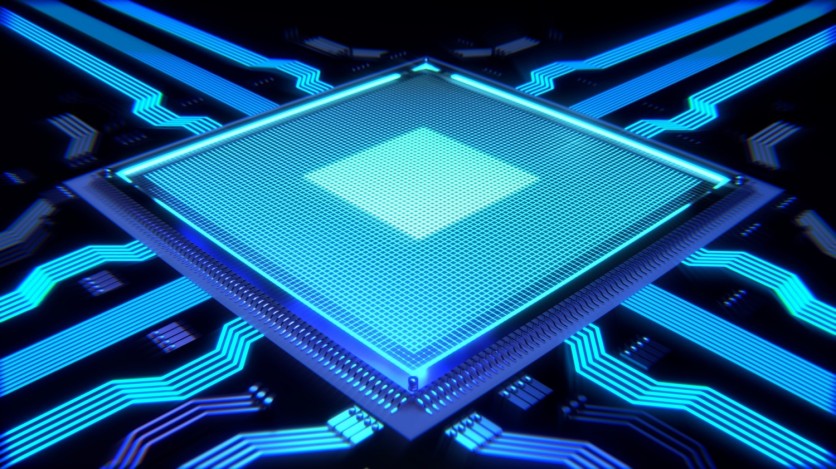
Fresh Approach to Machine Learning
This innovative study introduces a fresh approach to machine learning that prioritizes acquiring safe actions while striking a balance between optimality, encountering hazardous situations, and swiftly identifying unsafe acts.
Juan Andres Bazerque, an assistant professor in Electrical and Computer Engineering (ECE) at the Swanson School of Engineering, led this research in collaboration with Enrique Mallada, an associate professor in ECE at Johns Hopkins University.
Bazerque explained the fundamental difference between learning safe policies, and optimal policies, highlighting the potential pitfalls of solely pursuing optimized solutions in machine learning.
Recognizing the need for empirical evidence, the research team conducted comprehensive studies in two distinct scenarios to demonstrate the effectiveness of their approach.
By incorporating reasonable assumptions about exploration, they devised an algorithm capable of detecting all unsafe actions within a limited number of iterations. Additionally, the team addressed the challenge of finding optimal policies for a Markov decision process (MDP) with nearly certain constraints.
The examination emphasized the intricate balance between the duration needed to detect unsafe actions and the degree of exposure to dangerous circumstances.
Read Also : Researchers Develop Machine Learning Prescriptions and Reduce 'Antibiotic Resistance' By Half
Markov Decision Process
Within their research, the mathematical framework known as the Markov decision process (MDP) played an essential role in modeling decision-making affected by both chance and controlled variables.
In order to authenticate their theoretical discoveries, the scientists conducted thorough simulations that served to reaffirm the identified tradeoffs. These outcomes additionally uncovered the potential for accelerating the acquisition of knowledge and skills by incorporating safety constraints into the learning process.
Highlighting the significance of their study, Bazerque stated, "This research challenges the prevailing belief that learning safe actions requires an unlimited number of trials."
"Our results demonstrate that by effectively managing tradeoffs between optimality, exposure to unsafe events, and detection time, we can achieve guaranteed safety without an infinite number of explorations. This has significant implications for robotics, autonomous systems, and artificial intelligence, and more," Bazerque added.
As the pursuit of AI safety continues to evolve, this research opens up new avenues for enhancing the reliability and security of machine learning in complex environments.
By instilling machines with the ability to learn safe actions efficiently, researchers are driving forward the development of technologies that can safeguard human well-being while operating autonomously. The study's findings were published in the journal IEEE Transactions on Automatic Control.
Related Article : Biotech Startup Uses Machine Learning Algorithms to Predict the Progress of Cancerous Tumors | How Does it Work
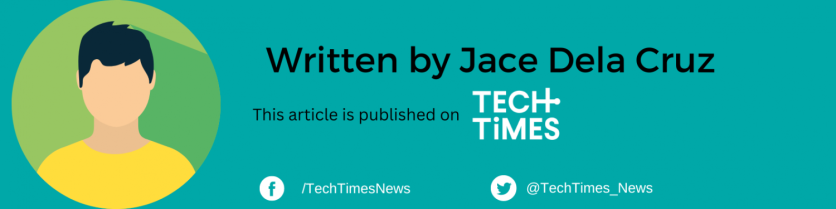
ⓒ 2025 TECHTIMES.com All rights reserved. Do not reproduce without permission.